Understanding Vector Norms in Statistics
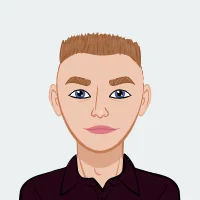
In the field of statistics, vector norms play a critical role in quantifying the size or magnitude of vectors, which are essential for various analytical techniques and models. Understanding vector norms is fundamental for students as it helps them measure distances between vectors, define convergence criteria, and implement regularization techniques in statistical modeling. This blog aims to provide a comprehensive exploration of vector norms, particularly focusing on weak q norms, to equip students with practical insights and skills applicable to assignments and real-world scenarios. Vector norms, including types such as L1, L2, and weak q norms, assign a non-negative scalar value to vectors, enabling analysts to compare and measure their sizes effectively. For assistance with understanding and applying these concepts effectively, students can benefit from consulting with experts at Statistics Homework Helper.
What are Vector Norms?
Vector norms serve as measurement tools to quantify the magnitude of vectors in statistical analysis. They are crucial for defining distances between vectors, which is foundational for tasks like clustering, classification, and regression analysis. The L1 norm, for example, measures the sum of the absolute values of vector components, making it robust against outliers and suitable for applications requiring feature selection in machine learning.
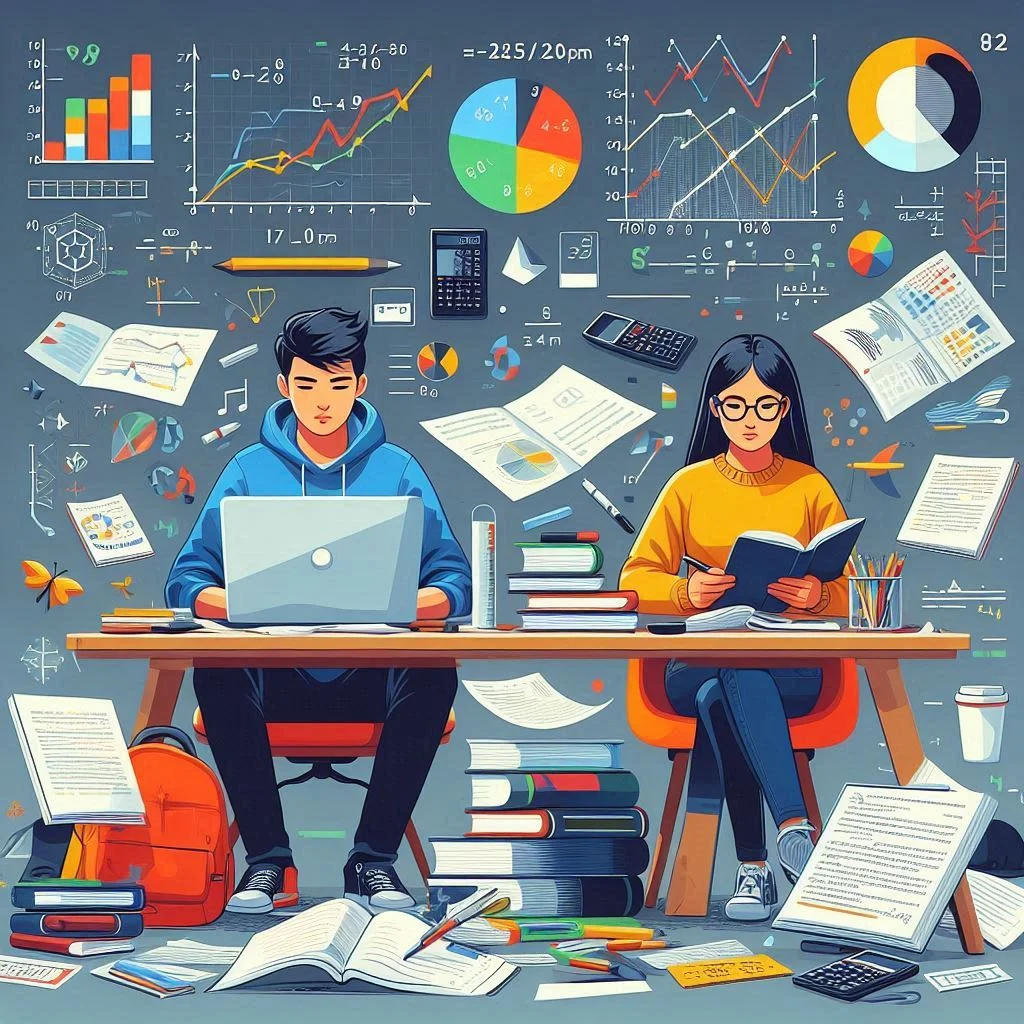
Vector norms serve as measurement tools to quantify the magnitude of vectors in statistical analysis. They are crucial for defining distances between vectors, which is foundational for tasks like clustering, classification, and regression analysis. The L1 norm, for example, measures the sum of the absolute values of vector components, making it robust against outliers and suitable for applications requiring feature selection in machine learning.
Similarly, the L2 norm calculates the Euclidean distance from the origin to the vector, which is commonly used in least squares problems and regularization techniques to ensure smooth and interpretable model solutions. Weak q norms extend these concepts by introducing a parameter q that adjusts the rate at which vector components contribute to the overall size, offering flexibility in measuring vector magnitudes based on varying decay rates.
Understanding vector norms empowers you to navigate complex assignments and research problems by providing you with foundational knowledge that bridges theory and practice in statistical analysis, helping you solve your statistics homework effectively.
Exploring Weak q Norms
Weak q norms are a specialized type of vector norm that adapts the decay rates of vector components based on the parameter q. This section explores how weak q norms are defined and their practical implications in statistical modeling and analysis. Unlike L1 and L2 norms, which have fixed decay rates for vector components, weak q norms provide a more flexible approach that accommodates varying rates of contribution among vector elements.
In statistical applications, weak q norms are particularly useful for tasks requiring controlled sparsity or regularization of model coefficients. By adjusting the parameter q, analysts can tailor the strength of regularization and balance between model complexity and predictive accuracy. This flexibility enhances the interpretability of statistical models and enables robust decision-making in data-driven contexts.
Properties and Applications of Weak q Norms
The properties of weak q norms contribute to their versatility in statistical analysis. For instance, these norms maintain convexity and offer connections to Lp norms, where for certain values of q, weak q norms can be bounded by Lp norms. This relationship underscores their utility in optimization problems and regularization techniques, ensuring stable and reliable solutions to statistical challenges.
Applications of weak q norms span various domains within statistics, from machine learning and data mining to signal processing and image reconstruction. In practice, these norms facilitate the identification of important features in high-dimensional data, support clustering algorithms that account for varying densities, and promote sparsity in model representations for enhanced interpretability.
By exploring the practical applications and theoretical underpinnings of weak q norms, students gain insights into their role in shaping statistical methodologies and advancing analytical capabilities in data science.
Examples and Case Studies
To illustrate the practical relevance of weak q norms, this section presents examples and case studies that highlight their application in real-world scenarios. Case studies will cover instances where analysts use weak q norms to solve specific problems in statistical analysis, demonstrating how these norms contribute to effective decision-making and model development.
Example 1: Regularization in Machine Learning
Consider a scenario where analysts apply weak q norms to regularize coefficients in a machine learning model, such as Lasso regression. By adjusting the q parameter, analysts can control the sparsity of model features, facilitating feature selection and promoting simpler, more interpretable models. This application of weak q norms enhances the model's robustness to noise and outliers, improving its generalization performance in predictive tasks.
Example 2: Clustering Analysis
In clustering analysis, weak q norms enable analysts to measure the similarity between data points based on varying rates of feature importance. By incorporating weak q norms into clustering algorithms, analysts can identify clusters with different densities and shapes, accommodating complex data structures more effectively than traditional norms. This capability supports accurate segmentation of data, facilitating targeted marketing strategies and personalized recommendations in various industries.
Through these examples and case studies, students gain practical experience in applying weak q norms to solve assignments and real-world challenges in statistical analysis. By examining how these norms are implemented in different contexts, students enhance their understanding of their applications and develop proficiency in leveraging them for effective data analysis and decision-making.
Comparing Weak q Norms with Other Norms
Comparative analysis between weak q norms and other commonly used norms, such as L1 and L2 norms, provides valuable insights into their respective strengths and applications in statistical analysis. While L1 norms emphasize sparsity and are robust to outliers, L2 norms prioritize smoothness and are commonly used in regression and optimization problems. In contrast, weak q norms offer a flexible framework that adjusts the decay rates of vector components, making them suitable for scenarios requiring nuanced control over model complexity and feature importance.
Comparison with L1 Norms
Weak q norms generalize L1 norms by allowing analysts to adjust the decay rates of vector components based on the parameter q. This flexibility enhances the robustness of statistical models by promoting sparsity and simplifying model interpretations. In contrast, L1 norms enforce strict sparsity by penalizing the absolute values of coefficients, facilitating feature selection and dimensionality reduction in high-dimensional data analysis.
Comparison with L2 Norms
Weak q norms differ from L2 norms in their treatment of vector magnitudes and decay rates. While L2 norms calculate the Euclidean distance from the origin to the vector, weak q norms adjust this distance based on the parameter q, allowing analysts to modulate the contributions of vector components. This capability supports optimization problems and regularization techniques that require balancing model complexity and predictive accuracy, ensuring stable and reliable solutions to statistical challenges.
By understanding these comparative aspects, students can make informed decisions when selecting norms for specific applications in statistical analysis. By recognizing the strengths and limitations of each type of norm, students can leverage their knowledge to develop effective solutions to assignments and research problems, demonstrating proficiency in applying advanced statistical techniques.
Practical Tips for Mastering Vector Norms
Achieving proficiency in vector norms in statistics requires a combination of theoretical understanding and practical application. This section offers actionable tips and strategies to help you enhance your skills in handling assignments and projects related to vector norms, with a particular focus on weak qqq norms. By applying these insights, you will be better equipped to tackle complex problems and successfully complete your statistics homework with greater confidence and accuracy.
Tip 1: Develop a Solid Theoretical Foundation
Start by mastering the definitions, properties, and practical implications of vector norms, including weak q norms. A solid theoretical foundation enables students to grasp the underlying principles that govern norm calculations and their applications in statistical analysis. Engaging with textbooks, online resources, and academic literature can provide comprehensive insights into vector norms and their role in modern data science.
Tip 2: Explore Real-World Applications
Apply theoretical knowledge to practical scenarios by working through case studies and examples that demonstrate the application of vector norms in statistical analysis. By engaging with real-world data and problem-solving exercises, students gain hands-on experience in using vector norms to address complex challenges in data science and machine learning. This practical approach enhances understanding and reinforces theoretical concepts, preparing students for assignments and research projects that require proficiency in vector norm calculations.
Tip 3: Experiment with Statistical Software
Utilize statistical software tools such as R, Python, or MATLAB to compute and visualize vector norms in practice. Experimenting with different parameter settings and datasets allows students to explore the impact of varying norms on model outcomes and performance metrics. By gaining proficiency in software tools, students develop practical skills that are essential for conducting data-driven analyses and presenting findings effectively.
By following these tips and strategies, students can enhance their proficiency in mastering vector norms, particularly focusing on weak q norms. By combining theoretical knowledge with practical application, students can effectively tackle assignments, research projects, and real-world challenges in statistical analysis, demonstrating their expertise and advancing their careers in data science and related fields.
Conclusion
In conclusion, understanding vector norms, including weak q norms, is essential for students pursuing careers in statistics and data science. By exploring the definitions, properties, and practical applications of vector norms, students gain valuable insights into their role in statistical analysis and decision-making. The ability to apply vector norms effectively in assignments and research projects enhances students' analytical skills and prepares them for success in academic and professional endeavors.
By mastering these concepts and leveraging practical tips for application, students can navigate complex data analysis tasks with confidence, contributing to advancements in statistical methodologies and data-driven insights. Vector norms serve as foundational tools that empower students to interpret data effectively, develop robust models, and make informed decisions based on sound statistical principles. As students continue to explore and apply vector norms in their studies and careers, they contribute to the evolution of statistical analysis and its applications across diverse industries and disciplines.