The Impact of Variance and Standard Deviation on Data Interpretation
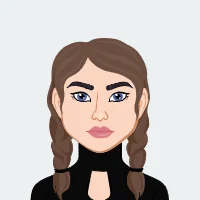
Statistics is an essential field with a profound impact on various academic disciplines and professional domains. Mastery of statistical methods is critical for students who aim to excel academically and make informed decisions based on data. Effectively tackling statistical assignments involves more than just applying formulas; it requires a deep understanding of core concepts and strategies. This blog aims to provide students with a comprehensive guide to navigate statistical assignments successfully, focusing on foundational elements such as variables, measures of location, random sampling, standard error, and confidence intervals.
By delving into these key concepts, students can gain a clearer understanding of how to approach their assignments with greater confidence and precision. Grasping the nature of different types of variables and how they influence data analysis is crucial for selecting appropriate statistical methods. Measures of location, including the mean, median, and mode, offer insights into the central tendencies of data, helping students summarize and interpret complex datasets effectively.
Understanding random sampling is vital for ensuring that a sample accurately represents the broader population, thereby minimizing bias and enhancing the generalizability of results. The standard error provides a measure of the precision of sample estimates, indicating how closely the sample mean reflects the population mean. Finally, confidence intervals offer a range within which the true population parameter is likely to fall, adding an extra layer of reliability to statistical conclusions. By mastering these concepts, students will be better equipped to tackle statistical assignments with greater accuracy, leading to more meaningful and impactful analysis. If you need additional support, expert help can guide you through these concepts and effectively solve your statistics homework, ensuring a thorough understanding and successful completion of your assignments.
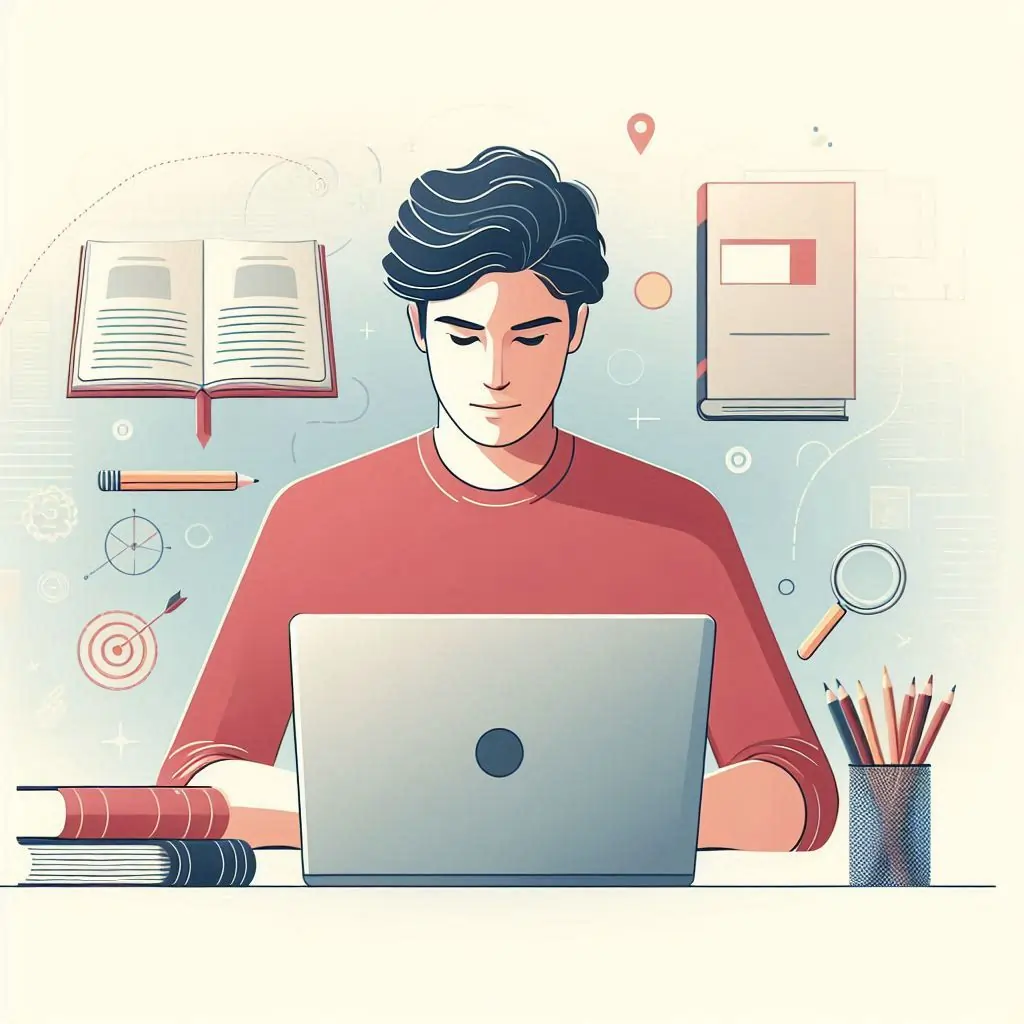
Understanding Variables in Statistical Analysis
Variables are the foundation of any statistical analysis. They represent the characteristics or attributes of the subjects being studied. In the context of the provided assignment, the variable of interest is the "distance walked per week" by visually impaired Dutch people. But what exactly is a variable, and why is it important?
Types of Variables
Variables can be broadly categorized into two types: quantitative and qualitative.
- Quantitative Variables: These variables represent numerical values and can be further divided into continuous and discrete variables. Continuous variables, like distance walked per week, can take any value within a given range. In contrast, discrete variables take on specific, distinct values, such as the number of children in a family.
- Qualitative Variables: These variables represent categories or attributes and are not numerical. For example, gender, eye color, and nationality are qualitative variables.
Understanding the type of variable you're working with is crucial because it determines the type of statistical analysis you can perform. In our case, "distance walked per week" is a continuous quantitative variable, which allows for a range of statistical methods to be applied.
Why Understanding Variables Matters
Recognizing the type of variable is important for several reasons:
- Choice of Analysis: The type of variable dictates the statistical methods you can use. For example, mean, median, and mode are commonly used for quantitative variables, while frequencies and proportions are more suitable for qualitative variables.
- Data Collection and Measurement: Understanding variables helps in designing surveys and experiments. For instance, knowing that "distance walked" is a continuous variable informs how you measure and record this data accurately.
- Interpretation of Results: Properly identifying variables ensures that the results of your analysis are interpreted correctly. Misidentifying a variable can lead to incorrect conclusions, which could compromise the validity of your findings.
Practical Tips for Students
- Clarify Variable Types: Always start by identifying whether your variables are quantitative or qualitative, and whether they are continuous or discrete. This will guide your analysis approach.
- Consult Your Assignment Requirements: Ensure that you understand the specific requirements of your assignment regarding the variables. This can help avoid common pitfalls and ensure that you are meeting the expectations of your instructor.
- Use Appropriate Graphical Tools: Utilize histograms, scatter plots, or box plots for quantitative variables and bar charts or pie charts for qualitative variables. These tools provide a visual representation of the data, making it easier to understand and analyze.
Understanding variables in statistical analysis is essential for mastering your assignments and ensuring you can effectively complete your statistical analysis homework. Variables define what is being measured and are classified as qualitative (categorical) or quantitative (numerical). Identifying the type of variable helps in choosing the appropriate analytical methods—qualitative variables might use frequency distributions or chi-square tests, while quantitative variables require measures of central tendency and dispersion. By accurately handling variables, you tailor your analysis to the data and meet assignment requirements, enhancing the accuracy, relevance, and impact of your findings. This leads to valid conclusions and effective communication of results.
Selecting Appropriate Measures of Location
Measures of location, also known as measures of central tendency, are statistical tools used to summarize a set of data by identifying the central point within that dataset. These measures include the mean, median, and mode, each providing different insights into the data distribution. In statistical assignments like the one provided, selecting the appropriate measure of location is crucial for accurately interpreting the data.
Key Measures of Location
- Mean: The mean, or average, is the sum of all data points divided by the number of data points. It is widely used because it considers every value in the dataset, making it sensitive to outliers.
- Median: The median is the middle value in a dataset when the values are arranged in ascending or descending order. It is particularly useful when the data is skewed, as it is not affected by extreme values.
- Mode: The mode is the value that appears most frequently in a dataset. It is the only measure of central tendency that can be used with nominal data, making it useful for qualitative data analysis.
Choosing the Right Measure
The choice of which measure of location to use depends on the characteristics of your data:
- Symmetrical Distribution: If the data is symmetrically distributed (i.e., the distribution is not skewed), the mean is often the best measure of central tendency because it uses all data points.
- Skewed Distribution: In cases where the data is skewed, the median is typically more informative because it is less influenced by outliers and skewed data.
- Multimodal Data: When your data has more than one mode (i.e., it is multimodal), the mode can provide insight into the most common values within the dataset.
Using Graphical Methods
Graphical methods are invaluable for visualizing the distribution of your data and selecting the appropriate measure of location:
- Histograms: A histogram is a bar graph that represents the frequency of data points within specified intervals. It can help you visualize the distribution of your data and identify whether it is symmetrical or skewed, which in turn guides your choice of the measure of central tendency.
- Box Plots: A box plot (or whisker plot) displays the distribution of data based on a five-number summary: minimum, first quartile, median, third quartile, and maximum. Box plots are useful for identifying outliers and understanding the spread of your data, which can influence your choice of the median or mean.
Practical Tips for Students
- Analyze Data Distribution: Before choosing a measure of location, analyze the distribution of your data using graphical methods like histograms or box plots.
- Consider Outliers: If your data contains outliers, consider using the median instead of the mean to reduce the impact of these extreme values.
- Report Multiple Measures: In some cases, it may be beneficial to report more than one measure of location to provide a more comprehensive summary of your data.
By understanding and selecting the appropriate measures of location, you can effectively summarize your data and provide meaningful insights in your statistical assignments.
The Significance of Random Sampling in Statistics
Random sampling is a fundamental concept in statistics, ensuring that the sample you collect accurately represents the population you are studying. In the context of the provided assignment, the importance of random sampling cannot be overstated, as it directly impacts the validity and reliability of the results.
What is Random Sampling?
Random sampling is a method where each member of a population has an equal chance of being selected for the sample. This process eliminates selection bias and ensures that the sample is representative of the entire population, allowing for generalizable conclusions.
Types of Random Sampling
There are several methods of random sampling, each with its own advantages:
- Simple Random Sampling: In simple random sampling, every member of the population has an equal chance of being selected. This method is straightforward but requires a complete list of the population, which can be challenging to obtain in large populations.
- Stratified Random Sampling: Stratified sampling involves dividing the population into subgroups (strata) based on specific characteristics, such as age or gender, and then randomly selecting samples from each stratum. This method ensures that different subgroups are adequately represented in the sample.
- Systematic Sampling: In systematic sampling, every nth member of the population is selected after choosing a random starting point. This method is easier to implement than simple random sampling but may introduce bias if there is a hidden pattern in the population list.
Why Random Sampling Matters
Random sampling is crucial for several reasons:
- Reduces Bias: By giving each member of the population an equal chance of selection, random sampling minimizes bias, ensuring that the sample is representative of the population.
- Enhances Validity: A random sample increases the validity of the results by reducing the likelihood that the findings are influenced by a non-representative sample.
- Facilitates Generalization: When a sample is randomly selected, the results can be more confidently generalized to the broader population, making the findings more applicable and meaningful.
Practical Application in the Assignment
In the provided assignment, random sampling was used to select 200 visually impaired Dutch people to study their walking habits. The significance of this random selection lies in its ability to ensure that the sample's walking habits reflect those of the entire population of visually impaired Dutch people, not just a biased subset.
Practical Tips for Students
- Understand the Sampling Method: Always clarify the sampling method used in your study. Understanding whether the sample was truly random is essential for interpreting the results accurately.
- Evaluate Sample Representativeness: Consider whether your sample is representative of the population. If the sample is not random, acknowledge this limitation and consider its impact on your findings.
- Use Random Sampling Tools: Utilize tools and software to ensure that your sampling process is truly random. This can include random number generators or specialized statistical software.
Random sampling is a cornerstone of robust statistical analysis. By ensuring that your sample is representative of the population, you can produce more reliable and valid results in your assignments.
The Role of Standard Error in Statistical Analysis
The standard error (SE) is a critical concept in statistics that measures the precision of a sample estimate. Understanding the standard error is essential for interpreting your statistical results and making informed conclusions about the population from which the sample was drawn.
What is Standard Error?
The standard error is the standard deviation of the sampling distribution of a statistic, most commonly the mean. It provides an estimate of the variability of the sample mean if you were to take multiple samples from the same population. The smaller the standard error, the more precise your sample mean is as an estimate of the population mean.
Why Standard Error Matters
The standard error plays a crucial role in several aspects of statistical analysis:
- Confidence Intervals: The standard error is used to calculate confidence intervals, which provide a range of values within which the population mean is likely to fall. A smaller standard error results in a narrower confidence interval, indicating more precise estimates.
- Hypothesis Testing: In hypothesis testing, the standard error is used to determine the significance of the results. A smaller standard error suggests that the sample mean is close to the population mean, increasing the likelihood that observed differences are statistically significant.
- Interpreting Results: Understanding the standard error helps in interpreting the reliability of your results. A larger standard error indicates greater variability and less confidence in the sample mean as an estimate of the population mean.
Practical Application in the Assignment
In the provided assignment, the standard error can be used to assess the reliability of the sample mean distance walked by the 200 visually impaired Dutch people. If the standard error is small, it indicates that the sample mean is a good estimate of the population mean, providing confidence in the results.
Practical Tips for Students
- Always Calculate the Standard Error: When reporting the mean of a sample, always include the standard error to provide a measure of precision.
- Use Standard Error to Inform Conclusions: Consider the standard error when interpreting your results. A small standard error suggests that your findings are robust and likely to reflect the true population values.
- Report Confidence Intervals: Alongside the standard error, report confidence intervals to provide a more complete picture of the uncertainty surrounding your estimates.
The standard error is a vital tool for assessing the precision of your statistical estimates. By understanding and correctly interpreting the standard error, you can enhance the credibility and accuracy of your statistical analysis.
Conclusion
Understanding foundational statistical concepts such as variables, measures of location, random sampling, and standard error is crucial for conducting accurate and insightful data analysis. Variables define what you measure and how you analyze it, and recognizing their types helps in selecting appropriate analytical methods. Measures of location, including the mean, median, and mode, provide a summary of central tendencies, distilling complex datasets into understandable figures and revealing underlying trends. Random sampling ensures that your sample represents the broader population accurately, minimizing bias and enhancing the generalizability of your results.
The standard error plays a critical role in assessing the precision of your sample estimates. A smaller standard error indicates that your sample mean is a more accurate reflection of the population mean, which strengthens the credibility of your findings. Reporting the standard error along with confidence intervals gives a fuller picture of the reliability and uncertainty of your results. Mastery of these concepts not only enhances the quality of your data analysis but also ensures that your conclusions are both reliable and impactful.