Understanding and Implementing Validation Studies in Data Analysis
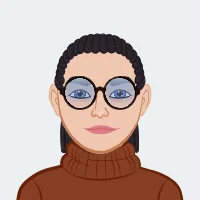
Validation studies are integral to assessing the reliability and relevance of measurement tools in statistics. They help determine how well a test or assessment correlates with or predicts relevant outcomes. This blog will guide you through the key aspects of validation studies, offering detailed insights into understanding and solving related assignments. Each section will explore different elements of validation studies, providing a comprehensive approach to tackling these complex assignments.
Introduction to Validation Studies
Validation studies are designed to evaluate the effectiveness of measurement tools, ensuring they accurately assess what they intend to measure. These studies are crucial for determining the validity of assessments, which can impact decisions ranging from academic evaluations to hiring processes. There are several types of validation studies, each serving a specific purpose:
- Predictive Validity: This type measures how well a test predicts future performance or behavior. For example, using a cognitive ability test to forecast job performance is assessing predictive validity. It involves collecting initial data and correlating it with future outcomes to determine how well the initial measures predict later performance.
- Concurrent Validity: Concurrent validity assesses how well a test correlates with a criterion measured simultaneously. For instance, comparing scores from a new performance evaluation tool with current performance metrics examines concurrent validity. This type of validation ensures that the tool is accurate in measuring the intended variables at the same time.
- Content Validity: Content validity evaluates whether a test covers the full scope of the domain it aims to measure. For example, a mathematics test should include questions on various topics within mathematics to ensure comprehensive coverage. This type of validity ensures that the assessment is representative of the entire content area.
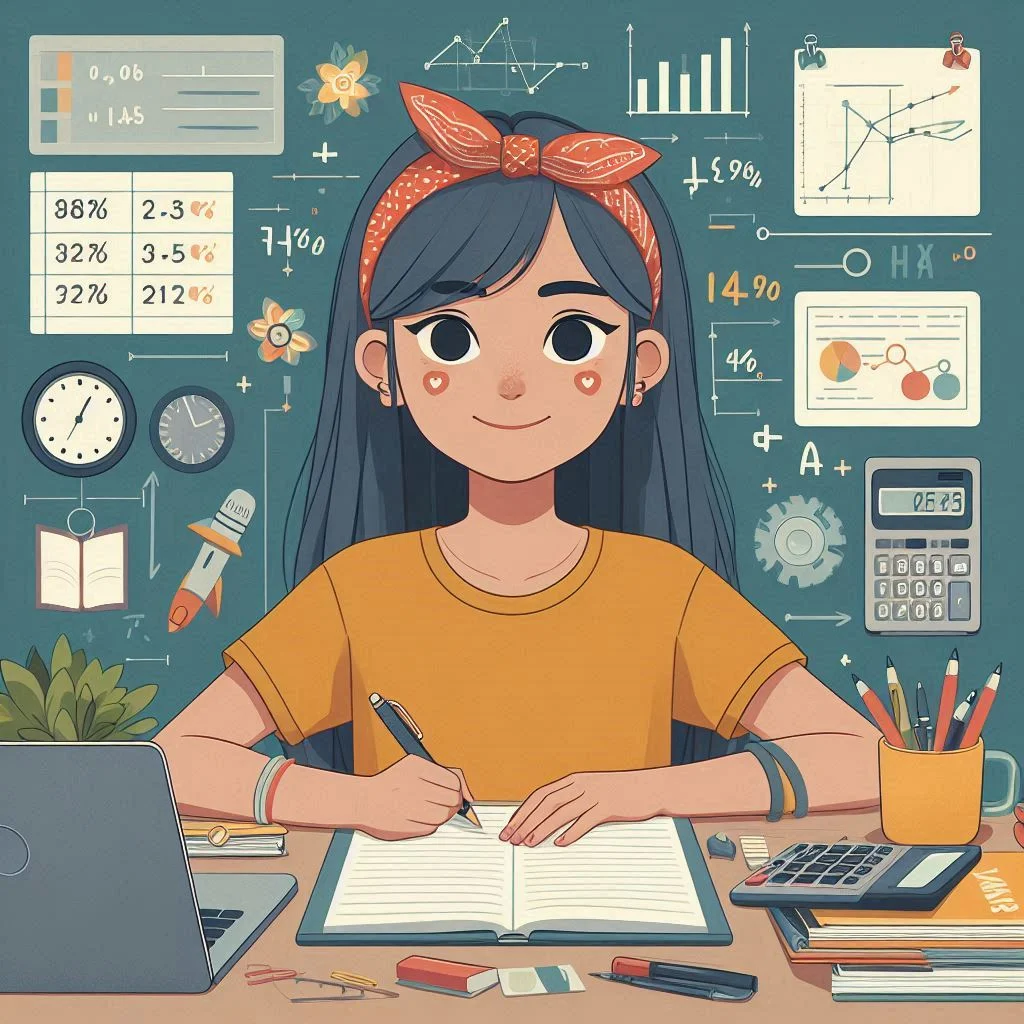
Understanding these types of validity helps in selecting the appropriate method for your validation study and applying it effectively to your assignments. If you’re looking to solve your data analysis homework, this foundational knowledge is crucial for analyzing how well measurement tools perform and making informed decisions based on your findings.
Analyzing the Dataset
Before diving into statistical analyses, it is essential to thoroughly understand your dataset. This involves familiarizing yourself with the variables and their measurement types:
- Dichotomous Variables: These variables have two possible outcomes, such as correct/incorrect or yes/no. In a dataset with cognitive ability assessments, dichotomous variables may indicate whether a response is correct (1) or incorrect (0). Accurately interpreting these variables is crucial for analyzing cognitive abilities.
- Likert Scale Variables: Likert scales are used to assess attitudes or behaviors on a scale, such as 1 to 7, where 1 represents strong disagreement and 7 represents strong agreement. Performance appraisal and organizational citizenship behavior (OCB) items often use this scale. It is important to handle these variables carefully, ensuring that responses are accurately recorded and interpreted.
- Timing of Data Collection: Consider when the data was collected. For instance, if cognitive ability was measured at hiring and performance appraisals and OCBs were measured six months later, you are conducting a predictive validity study. Understanding the timing helps in interpreting the relationships between variables and their predictive power.
By thoroughly analyzing the dataset and understanding the nature of each variable, you can ensure accurate and meaningful results in your validation study. This step is crucial for setting the stage for further analysis and interpretation.
Descriptive Statistics and Their Importance
Descriptive statistics summarize the basic features of your data, providing a clear overview of its central tendencies and variability. This foundational step is crucial for understanding the overall characteristics of your dataset:
- Calculating Average Age: To determine the mean age of the sample, sum all ages and divide by the number of participants. This provides a measure of central tendency, giving an overview of the age distribution in your sample.
- Gender Composition: Determine the percentage of men and women by counting the number of each gender, dividing by the total number of participants, and multiplying by 100. This helps in understanding the demographic makeup of your sample and ensures that it is representative of the population.
Descriptive statistics are essential for providing a summary of the dataset, allowing you to identify any trends or patterns. This information forms the basis for more complex analyses and helps in interpreting the results of your validation study.
Understanding Reliability and Its Impact
Reliability refers to the consistency and stability of a measurement tool. Ensuring that your data is reliable is crucial for accurate analysis and interpretation:
- Attenuation Due to Unreliability: Unreliability can weaken the observed relationships between variables. It is important to adjust for this by addressing any issues with reverse-coded items. Reverse-coded items should be recoded to ensure consistency in the scoring process.
- Handling Reverse-Coded Items: Identify any reverse-coded items in your dataset and adjust their scores accordingly. This ensures that high scores consistently reflect positive attributes, and low scores reflect negative attributes. Accurate handling of reverse-coded items is essential for maintaining the reliability of your measurements.
Understanding and addressing issues related to reliability ensures that your analysis accurately reflects the relationships between variables. This step is crucial for obtaining valid results and making informed decisions based on your findings.
Correlation Analysis: Measuring Relationships
Correlation analysis helps in understanding the relationships between variables by measuring the strength and direction of these relationships:
- Computing Bivariate Correlations: Use statistical software to calculate the correlation coefficients between cognitive ability, performance appraisal, and organizational citizenship behaviors (OCBs). These coefficients indicate how strongly each pair of variables is related. A positive correlation suggests that as one variable increases, the other also tends to increase, while a negative correlation indicates an inverse relationship.
- Interpreting Correlations: Analyze the correlation coefficients to understand the strength and direction of the relationships. For example, a strong positive correlation between cognitive ability and performance appraisal indicates that higher cognitive ability is associated with better performance. This information is crucial for assessing the validity of your measurement tools.
Correlation analysis provides insights into how variables are related and helps in understanding the dynamics between different aspects of your dataset. Accurate interpretation of correlations is essential for drawing meaningful conclusions from your validation study.
Variance Analysis and Its Implications
Analyzing variance helps in understanding how much one variable explains the variability in another. This is crucial for assessing the predictive power of variables:
- Variance in Performance Appraisal: Determine how much cognitive ability accounts for the variance in performance appraisal scores. This involves assessing the proportion of variability in performance appraisal that can be explained by cognitive ability.
- Variance in OCBs: Similarly, evaluate how cognitive ability explains the variance in OCB scores. This helps in understanding the predictive power of cognitive ability on organizational behaviors.
By analyzing variance, you can assess the extent to which one variable influences another and understand the implications for your validation study. This analysis is crucial for interpreting the effectiveness of measurement tools and making informed recommendations.
Correcting for Range Restriction
Range restriction can bias validity estimates if your sample does not fully represent the broader population. Correcting for this helps in obtaining more accurate estimates:
- Validity Adjustment: Adjust validity estimates to account for range restriction. This involves modifying your estimates to reflect the true relationship in a more representative or unrestricted sample. Range restriction occurs when the sample does not capture the full variability present in the general population.
- Understanding Range Restriction: Recognize that range restriction can lead to biased estimates if the sample is not representative of the broader population. Correcting for this helps in obtaining a more accurate estimate of the relationship between variables and ensures that your conclusions are valid.
Correcting for range restriction ensures that your validity estimates accurately reflect the true relationships between variables. This step is crucial for making informed decisions based on your analysis and improving the reliability of your results. By addressing range restriction, you can ensure that your findings are robust and applicable to broader contexts, helping you complete your statistics homework with confidence.
Interpreting Results and Making Recommendations
After completing your analysis, interpreting the results and making recommendations is the final step:
- Corrected Validity Estimates: Review the validity estimates after adjusting for reliability and range restriction. Determine whether the corrected estimates suggest that cognitive ability assessments are valuable for selection purposes. Consider the implications for hiring practices and whether the assessment adds value to the selection process.
- Decision Making: Based on the corrected validity estimates, decide if cognitive ability assessments should be used in selecting candidates. Assess the benefits and limitations of the assessment tool and make recommendations based on the results of your analysis.
Interpreting results and making recommendations involves evaluating the effectiveness of your measurement tools and determining their practical applications. This final step is crucial for applying your findings to real-world scenarios and making informed decisions based on your validation study.
Conclusion
Mastering validation studies in statistics requires a thorough understanding of various principles and methods. By following this comprehensive guide, you can approach your assignments with confidence, ensuring accurate and meaningful analysis. From understanding the types of validation studies to handling descriptive statistics, reliability, and range restriction, each step is crucial for effective analysis. With practice and attention to detail, you’ll be well-equipped to handle similar assignments and make informed decisions based on your statistical analyses.