Structured Procedures for MANOVA Assignment Completion
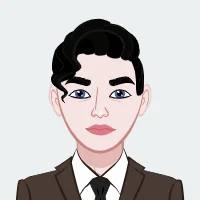
Multivariate Analysis of Variance (MANOVA) is an advanced statistical technique used to analyze the impact of one or more independent variables on multiple dependent variables simultaneously. Unlike univariate analysis, which examines the effect of independent variables on a single dependent variable, MANOVA allows researchers to investigate complex interactions and relationships between variables. This technique is particularly useful in fields such as psychology, education, and business, where multiple outcomes are of interest.
For students tackling complex assignments, understanding how to apply MANOVA can be crucial. If you are looking to solve your statistics homework effectively, mastering MANOVA is an essential skill. The primary advantage of MANOVA is its ability to handle multiple dependent variables, which provides a more holistic view of the data. For example, in a study examining the effect of accidents on job satisfaction and perceived risk, MANOVA can simultaneously assess how accident status influences both outcomes. This is advantageous over separate analyses that may not account for potential correlations between the dependent variables.
To effectively conduct a MANOVA analysis, it is crucial to understand and test several assumptions, perform the analysis correctly using statistical software like SPSS, interpret the results accurately, and report the findings in a structured manner. This blog will guide you through these steps, offering practical advice and examples to help you tackle MANOVA assignments with confidence and solve your statistics homework efficiently.
-Assignments.webp)
Testing Assumptions for MANOVA
Testing assumptions is a critical step in MANOVA to ensure the validity of the results. MANOVA relies on several key assumptions, and verifying these assumptions is essential to obtain reliable and accurate results. The primary assumptions for MANOVA include:
- Sample Size Considerations: A sufficient sample size is crucial for the reliability of MANOVA results. Larger sample sizes increase the power of the statistical tests, reducing the likelihood of Type II errors. In practice, aim for at least 20-30 cases per group, though this may vary based on the complexity of the model and the number of dependent variables.
- Equal Sample Sizes: While MANOVA can handle some imbalance in sample sizes, significant discrepancies can affect the robustness of the results. Unequal sample sizes can inflate Type I error rates and reduce statistical power. Techniques such as bootstrapping can be used to address imbalances and improve result stability.
- Multivariate Normality: MANOVA assumes that the dependent variables are jointly normally distributed within each group. This assumption is crucial for valid results. Assess multivariate normality using graphical methods (e.g., Q-Q plots) and statistical tests (e.g., Mardia’s test). If normality is violated, consider data transformations or alternative methods.
- Outliers: Outliers can significantly impact MANOVA results by distorting the analysis. Identify outliers using graphical methods (e.g., box plots) and statistical measures (e.g., Mahalanobis distance). Analyze the impact of outliers on your results and decide whether to include or exclude them based on their influence.
- Homogeneity of Covariance Matrices: This assumption requires that the variance-covariance matrices of the dependent variables are equal across groups. Use Box’s M test to assess this assumption. If violated, consider alternative methods or adjustments to account for differences in covariance matrices.
Understanding and testing these assumptions will help ensure that your MANOVA analysis is valid and reliable, providing accurate insights into the relationships between your variables.
Performing MANOVA in SPSS
Once the assumptions are tested and verified, you can proceed with performing MANOVA using SPSS. Here’s a step-by-step guide to conducting MANOVA in SPSS:
- Data Preparation: Ensure your data is correctly organized and cleaned before running MANOVA. Each row should represent a case, and each column should correspond to a variable. Handle missing values appropriately and ensure all variables are accurately labeled. For example, if your dataset includes variables such as accident status, risk level, and job satisfaction, verify that these variables are correctly coded.
- Accessing MANOVA in SPSS: Open SPSS and load your dataset. Navigate to Analyze > General Linear Model > Multivariate. This will open the MANOVA dialog box.
- Setting Up the Analysis: In the MANOVA dialog box, move your dependent variables (e.g., job satisfaction and perceived risk) to the “Dependent Variables” box. Move your independent variable (e.g., accident status) to the “Fixed Factor(s)” box. Click on the “Options” button to configure additional settings such as descriptive statistics, effect sizes, and post-hoc tests.
- Running the Analysis: Click “OK” to run the MANOVA analysis. SPSS will generate output including several tables and statistics. Review these tables to interpret the results. Key outputs include multivariate tests, univariate tests, and descriptive statistics.
- Saving and Exporting Results: After running the analysis, save your SPSS output file and export any relevant tables and figures for inclusion in your report. Ensure that all tables are clearly labeled and include relevant statistical information.
By following these steps, you can effectively perform MANOVA in SPSS and obtain the necessary results for interpretation and reporting.
Interpreting MANOVA Results
Interpreting the results from a MANOVA analysis involves examining both multivariate and univariate statistics to understand the effects of independent variables on the dependent variables. Here’s how to interpret the key results:
- Multivariate Tests: Begin with the multivariate significance tests, which provide an overall assessment of whether the independent variables significantly impact the dependent variables. Key statistics include Wilks’ Lambda, Pillai’s Trace, Hotelling’s Trace, and Roy’s Largest Root. Each statistic tests different aspects of the multivariate data:
- Wilks’ Lambda: Tests the null hypothesis that the means of the dependent variables are equal across groups. A significant Wilks’ Lambda indicates that at least one independent variable has a significant effect on the dependent variables.
- Pillai’s Trace: Measures the proportion of variance in the dependent variables explained by the independent variables. It is robust to assumption violations and provides an alternative to Wilks’ Lambda.
- Hotelling’s Trace: Tests whether the mean vectors of the dependent variables are equal across groups. Useful when sample sizes are equal and assumptions are met.
- Roy’s Largest Root: Focuses on the largest eigenvalue and tests whether there is a significant difference in the means of the dependent variables.
- Univariate Tests: Next, examine the univariate tests for each dependent variable. These tests evaluate the effect of the independent variables on each dependent variable individually. Look at the F-values and p-values for each dependent variable to identify significant effects. For instance, if the accident status significantly affects job satisfaction but not perceived risk, this indicates a notable impact on job satisfaction but not on perceived risk.
- Effect Sizes: In addition to statistical significance, consider effect sizes to assess the magnitude of the effects. Effect sizes provide a measure of the strength of the relationships between variables. Common effect size measures include partial eta squared and Cohen’s d. Reporting effect sizes helps contextualize the practical significance of your findings.
- Post-hoc Tests: If your MANOVA results indicate significant effects, consider conducting post-hoc tests to explore these effects further. Post-hoc tests compare group means to identify which specific groups differ from one another. This can provide additional insights into the nature of the relationships between variables.
By carefully interpreting these results, you can draw meaningful conclusions about the effects of independent variables on multiple dependent variables and better understand the underlying relationships in your data.
Reporting MANOVA Results
Reporting MANOVA results requires presenting your findings in a clear and structured format, typically following APA guidelines. Here’s how to effectively report your MANOVA results:
- Introduction: Begin with an introduction that provides context for the MANOVA analysis. Describe the research question, the purpose of the analysis, and the hypotheses being tested. For example, explain why it is important to examine the effect of accidents on job satisfaction and perceived risk and how MANOVA helps address these questions.
- Methods: In the methods section, detail the sample size, variables, and procedures used in the analysis. Include information about data collection, preprocessing steps, and criteria for including cases. Describe how you tested the assumptions and any adjustments made to address violations. Provide a clear overview of the MANOVA procedure, including how the analysis was set up in SPSS.
- Results: Present the results in a clear and organized manner. Use tables and figures to support your findings and report key statistics such as test statistics (e.g., Wilks’ Lambda), degrees of freedom, p-values, and effect sizes. Ensure that the results are easy to interpret and accurately reflect the findings from SPSS. Include both multivariate and univariate results and provide a comprehensive overview of the key findings.
- Discussion: In the discussion section, interpret the results in relation to the research question. Explain the implications of the findings and how they relate to the hypotheses. Address any limitations of the study, such as assumptions that were violated or potential biases in the data. Suggest directions for future research based on your findings. Discuss the practical significance of the results and their implications for the field.
By following these reporting guidelines, you can effectively communicate your MANOVA results and provide a comprehensive analysis that is informative and accessible to your audience.
Addressing Common Issues in MANOVA
During the analysis, you may encounter various challenges that need to be addressed to ensure the validity and reliability of your MANOVA results. Common issues include:
- Handling Assumption Violations: If assumptions are violated, consider alternative methods or adjustments to your analysis. For example, if multivariate normality is not met, you might use data transformations or consider alternative non-parametric tests. If homogeneity of covariance matrices is violated, use robust statistical methods or adjust your model to account for the differences.
- Interpreting Complex Results: MANOVA can produce complex results that may be difficult to interpret. If you encounter challenges in interpreting the results, seek assistance from statistical experts or use additional resources to clarify the findings. Consider using visualization techniques to help interpret complex relationships between variables.
- Dealing with Missing Data: Missing data can impact the validity of MANOVA results. Use appropriate methods to handle missing data, such as imputation or data augmentation. Ensure that missing data does not bias your results and that the methods used are suitable for your analysis.
- Reporting and Communication: Ensure that your reporting is clear and accurate, addressing all relevant aspects of the MANOVA analysis. Provide detailed explanations of your methods, results, and interpretations to help readers understand your findings. Avoid jargon and technical language that may confuse readers.
- Software and Technical Issues: Technical issues with software or data can impact your analysis. Ensure that you are using the latest version of statistical software and that your data is properly formatted. Address any technical problems promptly to avoid impacting your results.
By addressing these common issues, you can enhance the quality and reliability of your MANOVA analysis and ensure that your findings are robust and meaningful.
Conclusion
Approaching MANOVA assignments involves understanding the underlying principles, testing assumptions, performing the analysis in statistical software, interpreting the results, and reporting findings accurately. To effectively complete your Statistics homework, it is essential to follow a structured approach and address common challenges. By mastering these aspects, you can effectively tackle MANOVA assignments and provide valuable insights into complex relationships between variables. With the knowledge and skills acquired from this guide, you can approach MANOVA assignments with confidence and contribute to meaningful research in your field. Whether you are analyzing data for academic purposes or practical applications, a thorough understanding of MANOVA will enhance your ability to draw accurate conclusions and make informed decisions based on your analysis.