Precision Techniques for Bivariate and Multiple Regression Using SPSS
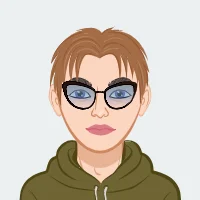
Regression analysis is an essential statistical method used to understand the relationships between different variables and to predict outcomes based on these relationships. In academic settings, particularly in statistics and research-oriented courses, regression analysis is frequently employed to explore how various independent variables influence a dependent variable. This blog aims to provide a comprehensive guide to performing bivariate and multiple regression analyses using SPSS, using a hypothetical assignment scenario as an example. By understanding and applying the principles outlined here, students can effectively tackle similar assignments and enhance their analytical skills. For students needing help with statistics homework using SPSS, this guide will serve as a valuable resource.
The hypothetical assignment involves using data on several variables: gender, eye color, the value of the gift given to the instructor, ERMA 7300 final grades, GRE Quantitative Scores, and ERMA 7310 final grades. The primary goal is to predict the ERMA 7310 final grade, which is the dependent variable, based on various predictor variables through both bivariate and multiple regression analyses.
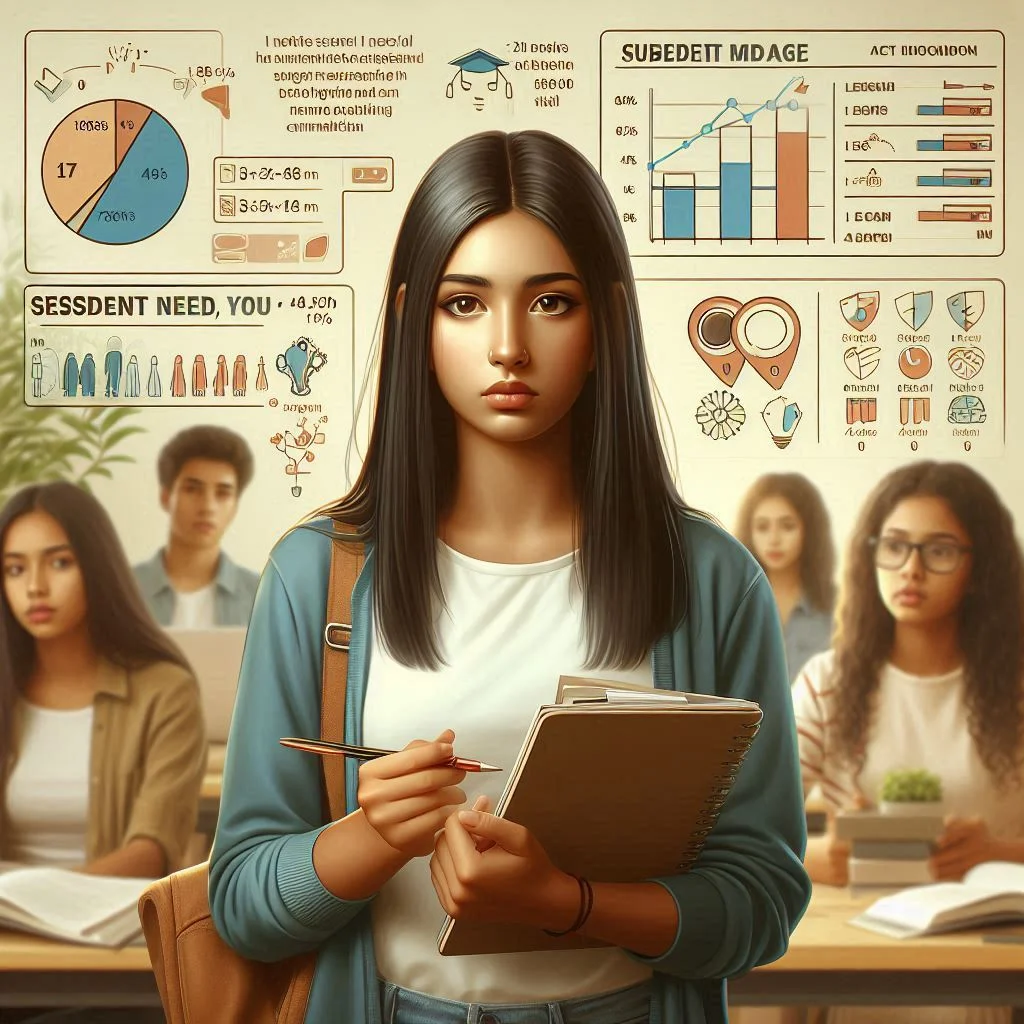
The process begins with preparing the data in SPSS, which includes entering or importing data, checking for any anomalies, and ensuring that the data meets the necessary assumptions for regression analysis. Once the data is ready, the next step is to perform bivariate regression analyses, where each predictor variable is individually analyzed to see how well it predicts the ERMA 7310 grade.
After identifying the best predictor through bivariate analysis, multiple regression analysis is conducted using two methods: forced entry and backward elimination. These methods help in understanding the combined effect of all predictor variables on the dependent variable. The final step involves comparing the models from both methods to select the most effective regression equation.
By following these steps, students can gain a deeper understanding of regression analysis and its applications, enabling them to confidently approach and solve similar statistical assignments. This guide will help you solve your statistics homework with clarity and precision.
Preparing the Data in SPSS
Before performing any regression analysis, it's crucial to prepare your data correctly in SPSS. Proper data preparation ensures that your analysis will be accurate and reliable, free from errors that could skew the results.
Data Entry and Preparation
The first step is to enter or import your data into SPSS. Ensure that each variable, such as gender, eye color, value of the gift, ERMA 7300 grades, GRE Quantitative Scores, and ERMA 7310 grades, is correctly labeled. This makes it easier to identify and work with each variable during the analysis. Clean your data by checking for any missing values or outliers, which can significantly impact your regression results. If you find any, decide whether to remove them or replace them with appropriate values.
Assumption Testing
Regression analysis relies on several key assumptions: linearity, independence, homoscedasticity, and normality of residuals. Linearity means that the relationship between the independent and dependent variables should be linear. Independence implies that the observations are independent of each other. Homoscedasticity indicates that the variance of the residuals (errors) is constant across all levels of the independent variables. Normality of residuals means that the residuals should be normally distributed. Checking these assumptions helps validate your data and ensures the reliability of your regression model.
Descriptive Statistics and Initial Checks
Before jumping into regression analysis, it's beneficial to run some descriptive statistics and initial checks. Descriptive statistics provide a summary of your data, including means, medians, standard deviations, and ranges. Initial checks, such as correlation matrices, can help you understand the relationships between your variables and identify any potential multicollinearity issues. If you want to solve your statistics homework using SPSS, understanding these preparatory steps is crucial.
Performing Bivariate Regression
Bivariate regression involves analyzing the relationship between two variables: one independent and one dependent. This type of analysis helps in understanding how changes in the independent variable affect the dependent variable.
Setting Up Bivariate Regressions
In SPSS, go to the Analyze menu, select Regression, and then Linear. Set your dependent variable as the ERMA 7310 final grade. For each bivariate regression, use one of the predictor variables (Gender, Gift Value, GRE Quantitative Scores, ERMA 7300 grades). This will help you generate four different bivariate regression equations. Each equation will show how one predictor variable affects the dependent variable.
Interpreting Bivariate Results
For each bivariate regression, look at the regression equation provided by SPSS. This equation helps you understand how each predictor variable affects the ERMA 7310 grades. Check the proportion of variance explained (R-squared value) to see how much of the variability in ERMA 7310 grades is accounted for by each predictor. Use these equations to make predictions for hypothetical students based on the given predictor values. For instance, you can predict the ERMA 7310 grade for a student who is male, gave a gift worth $50, has a GRE Quantitative Score of 475, and earned a final ERMA 7300 grade of 78.
Assessing Predictor Variables
It's important to assess the significance of each predictor variable in your bivariate regression models. SPSS provides significance values (p-values) that indicate whether the relationship between the predictor and the dependent variable is statistically significant. A predictor variable with a low p-value (typically less than 0.05) significantly affects the dependent variable. This assessment helps you understand which predictors are worth considering in further analysis.
Identifying the Best Predictor
After performing bivariate regressions, the next step is to identify which predictor variable is the most effective in predicting the dependent variable. This process involves comparing the results from each bivariate regression to determine the best predictor.
Comparing Bivariate Regressions
After running all four bivariate regressions, compare the R-squared values to determine which predictor variable explains the most variance in ERMA 7310 grades. The predictor with the highest R-squared value is considered the best predictor. This step helps you understand which variable has the most significant impact on the outcome. Additionally, consider the significance levels of the predictors to ensure that the best predictor is not only the one that explains the most variance but is also statistically significant.
Practical Implications
Understanding the best predictor can have practical implications for academic or professional settings. For example, if you identify that GRE Quantitative Scores are the best predictor of ERMA 7310 grades, you might focus more on improving students' GRE preparation. In academic research, identifying key predictors helps in formulating strategies and interventions that can lead to better outcomes.
Limitations and Considerations
While identifying the best predictor is valuable, it's also important to recognize the limitations of bivariate analysis. Bivariate regressions do not account for the combined effect of multiple predictors, which can provide a more comprehensive understanding of the factors influencing the dependent variable. Therefore, while the best predictor gives initial insights, further analysis through multiple regression is necessary for a more detailed understanding.
Performing Multiple Regression
Multiple regression analysis involves using more than one predictor variable to predict a dependent variable. This type of analysis helps in understanding the combined effect of multiple variables on the dependent variable.
Forced Entry Method
To perform a multiple regression, go to Analyze > Regression > Linear. Set ERMA 7310 final grade as the dependent variable and include all predictor variables (Gender, Gift Value, GRE Quantitative Scores, ERMA 7300 grades) as independent variables. Make sure the method is set to Enter. This will give you a comprehensive regression model that includes all predictors. The forced entry method is straightforward and includes all specified predictors in the model, allowing you to see the full effect of each variable.
Backward Elimination Method
In SPSS, you can also use the backward elimination method by selecting it in the regression options. This method starts with all predictors and iteratively removes the least significant ones. The final model will only include predictors that significantly contribute to predicting the ERMA 7310 grades. Backward elimination helps in simplifying the model by retaining only the most significant predictors, which can enhance the model's interpretability and predictive accuracy. If you need help with statistics homework using SPSS, mastering these methods is essential.
Interpreting Multiple Regression Results
For each multiple regression model, examine the regression coefficients, R-squared values, and significance levels. The regression coefficients indicate the strength and direction of the relationship between each predictor and the dependent variable. The R-squared value shows the proportion of variance in the dependent variable explained by the predictors. Significance levels help identify which predictors are statistically significant. This comprehensive analysis provides a deeper understanding of how multiple predictors interact to influence the dependent variable.
Comparing Multiple Regression Models
Once you have performed multiple regression analysis using both the forced entry and backward elimination methods, the next step is to compare the models to select the most effective one.
Evaluating and Selecting the Best Model
After running both the forced entry and backward elimination regressions, compare the models by looking at their R-squared values and the significance of the predictors. Use the F Change test in SPSS to compare the models statistically. The F Change test helps determine if the addition or removal of predictors significantly improves the model. Select the model that provides the best fit with the most significant predictors. This comparison helps you choose the most effective model for predicting ERMA 7310 grades. If you're looking to solve your statistics homework, understanding these comparisons is crucial.
Practical Considerations
When selecting the best model, consider not only the statistical measures but also the practical implications of the predictors. A model with significant and interpretable predictors is more valuable for practical applications. For example, a model that includes easily measurable predictors like GRE Quantitative Scores and previous grades might be more practical to implement than one that relies on less tangible predictors.
Model Validation
It's also essential to validate your selected model using a separate dataset or cross-validation techniques. This step ensures that the model generalizes well to new data and is not overfitted to the current dataset. Validation increases the reliability and credibility of your regression analysis, making your findings more robust and applicable in real-world scenarios.
Practical Tips for Solving Similar Assignments
Here are some practical tips to help you tackle similar assignments effectively and efficiently.
Understand the Assignment Requirements
Carefully read the assignment guidelines and understand what is required. Make sure you know which variables you need to analyze, what types of regression models you need to perform, and the specific outputs you are expected to provide. Understanding the requirements fully ensures that you can plan and execute your analysis correctly from the start.
Use SPSS Efficiently
Familiarize yourself with SPSS and its various functions for data preparation, assumption testing, and regression analysis. Learning how to use SPSS efficiently can save you time and help you avoid common pitfalls. If you need help with statistics homework using SPSS, there are many online tutorials and resources available to guide you through the process.
Check Assumptions Thoroughly
Before performing regression analysis, always check the assumptions of regression thoroughly. This includes checking for linearity, independence, homoscedasticity, and normality of residuals. Ensuring that your data meets these assumptions is crucial for obtaining valid and reliable results.
Document Your Process
Keep detailed notes and document each step of your analysis process. This includes data preparation, assumption testing, running regressions, and interpreting results. Clear documentation helps you keep track of your work and makes it easier to review and report your findings. Additionally, well-documented analysis can be a helpful reference for future assignments.
Seek Help When Needed
If you encounter difficulties or uncertainties, don't hesitate to seek help. Consulting with peers, instructors, or online resources can provide valuable insights and assistance. For more complex issues, consider reaching out to a tutor or professional who specializes in statistical analysis and SPSS. Solving your statistics homework becomes much more manageable with the right support.
Stay Organized
Organize your data, notes, and SPSS outputs in a systematic manner. Keeping everything organized helps you avoid confusion and makes it easier to locate specific information when needed. An organized approach streamlines your workflow and enhances the overall quality of your analysis.
Conclusion
By following these steps, you can effectively tackle assignments involving regression analysis using SPSS. Understanding how to perform and interpret both bivariate and multiple regressions will enhance your analytical skills and help you make informed predictions based on your data. Whether for academic projects or research, mastering these techniques is a valuable asset.
Regression analysis is a powerful tool for understanding relationships between variables and making predictions. By preparing your data properly, performing thorough analyses, and carefully interpreting your results, you can gain valuable insights and produce reliable findings. This comprehensive approach ensures that you are well-equipped to handle complex statistical assignments and contribute to your field with robust analyses and informed conclusions.