The Spatial Median: An Essential Concept in Multivariate Statistics
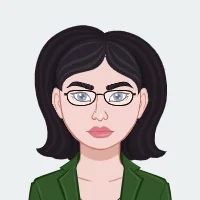
When delving into the world of statistics, one often begins with the basics of central tendency, such as exploring the mean and median. While the median is straightforward in one dimension, extending this concept to higher dimensions introduces us to the intriguing concept of the spatial median. This blog aims to provide you with a comprehensive understanding of the spatial median and its applications in multivariate statistics. Whether you're navigating through homework or seeking to deepen your knowledge in statistical analysis, grasping the spatial median can significantly enhance your proficiency. Understanding this concept not only equips you with essential tools for data interpretation but also empowers you to approach complex statistical problems with confidence, making it an invaluable resource in your academic journey. For expert guidance and assistance with your statistics homework, consider leveraging Statistics Homework Help to ensure accurate and insightful analysis.
Understanding the Spatial Median
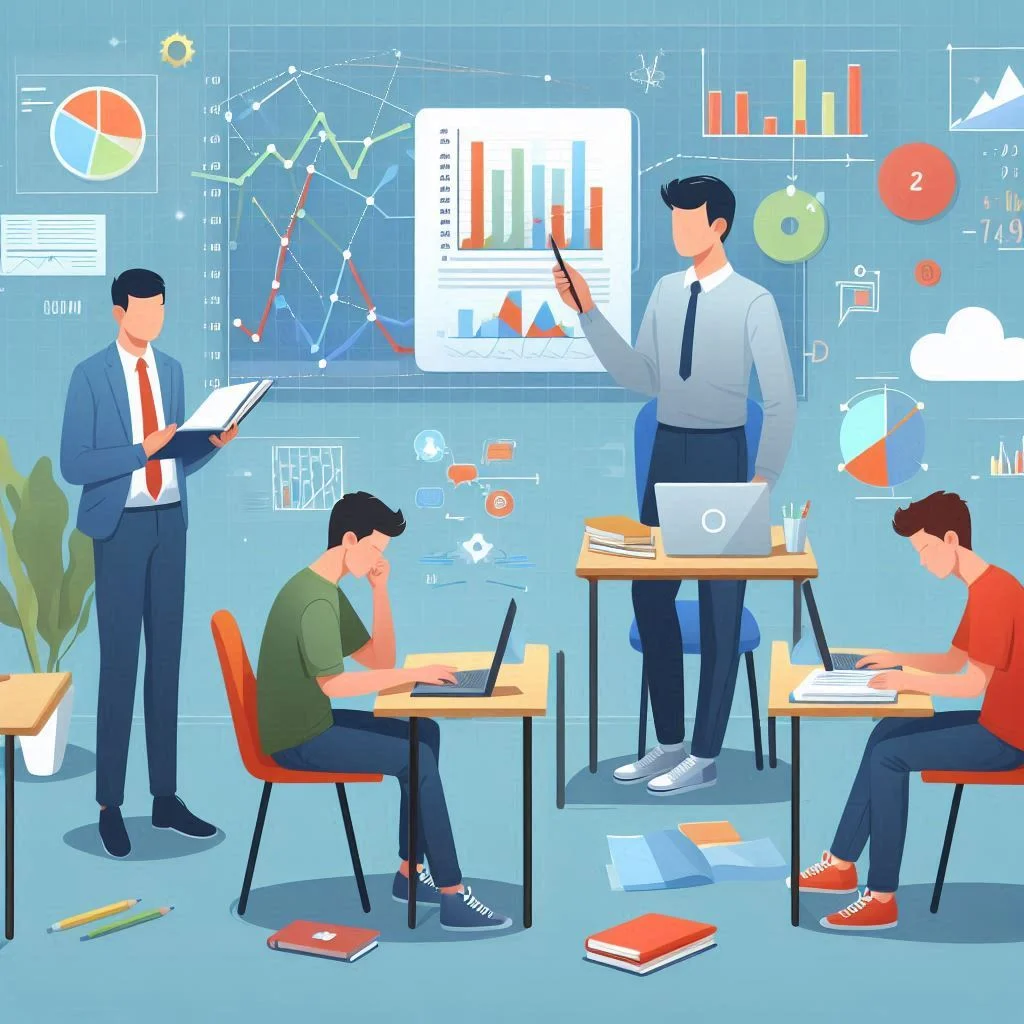
The spatial median is a generalization of the one-dimensional median into multiple dimensions. In a single dimension, the median minimizes the sum of absolute deviations from a central point. This concept can be extended to higher dimensions, where the spatial median represents a point that minimizes the overall distance to a set of points in a multidimensional space. This makes it an essential tool in multivariate statistics, helping to identify the central point in a dataset that is not confined to a single line or axis.
Defining the Spatial Median in Higher Dimensions
In higher dimensions, we use the Euclidean norm to define distances. The spatial median can be understood as the point that minimizes the total distance to all other points in a given dataset. For a probability measure in a multidimensional space, the spatial median is the point where this total distance is minimized. This concept is crucial for analyzing data that is spread across multiple dimensions, such as geographical data points, multivariate time series, or any other form of multidimensional statistical analysis.
Properties of Convex Sets and Convex Functions
A set is called convex if, for any two points within the set, the line segment connecting them also lies within the set. This property is vital when discussing the spatial median, as it ensures that the median lies within the bounds of the data. Additionally, a function defined on a convex set is called convex if the value of the function at any point on the line segment connecting two points is less than or equal to the weighted average of the function's values at those points. This property helps in minimizing functions to find the spatial median, as convex functions have well-defined minima.
Convexity and Strict Convexity in Functions
Convexity plays a significant role in determining the spatial median. A function is strictly convex if the function value at any point on the line segment between two points is strictly less than the weighted average of the function's values at those points. This property ensures uniqueness in the solution, which is essential for the spatial median. For example, a quadratic function is strictly convex, while the absolute value function is convex but not strictly convex. Understanding these properties helps in identifying the spatial median, as strictly convex functions have a unique minimum.
The Uniqueness and Existence of the Spatial Median
One of the key properties of the spatial median is its existence and uniqueness. For any probability measure in a multidimensional space, a spatial median always exists and does not depend on the initial point chosen. This property ensures that a central point can always be found within the dataset. Furthermore, if the data is not concentrated along a single line, the spatial median is unique. This uniqueness property is particularly useful in higher dimensions, where the spatial median provides a robust measure of central tendency that is less sensitive to outliers compared to the mean.
Applications of the Spatial Median in Statistics
The spatial median has numerous applications in statistics and data analysis. It is particularly useful in robust statistics, where it provides a measure of central tendency that is less affected by outliers and skewed distributions. The spatial median is also used in clustering algorithms, where it helps in finding the central point of clusters in a multidimensional space. Additionally, it is used in image processing, where it helps in reducing noise and identifying central features in images. Understanding these applications can help students in solving similar homework and applying the concept of the spatial median in various statistical analyses.
Solving Homework Using the Spatial Median
To solve spatial median homework, it is essential to understand the underlying principles and methodologies. Start by identifying the dataset and the dimensions involved. Next, apply the concept of minimizing the total distance to find the spatial median. Ensure that the dataset is well-defined and not concentrated along a single line to guarantee the uniqueness of the spatial median. Utilize convexity properties to simplify calculations and ensure accurate results. By following these steps and understanding the theoretical background, students can effectively tackle homework involving the spatial median and apply this knowledge to various statistical problems.
Benefits of Using the Spatial Median in Data Analysis
One of the significant benefits of using the spatial median in data analysis is its robustness. Unlike the mean, the spatial median is less affected by outliers and extreme values, providing a more accurate measure of central tendency in datasets with irregular distributions. This robustness makes it an ideal choice for analyzing real-world data, which often contains anomalies and outliers. Additionally, the spatial median is invariant under Euclidean transformations, such as rotations and translations, making it a reliable and consistent measure in various analytical contexts.
Practical Tips for Working with the Spatial Median
When working with the spatial median, it is important to consider the computational complexity, especially in high-dimensional datasets. Efficient algorithms and optimization techniques can help in finding the spatial median quickly and accurately. Software tools and statistical packages often include functions for calculating the spatial median, making it easier for students to implement this concept in their homework. Additionally, visualizing the data and the spatial median can provide valuable insights, helping to understand the distribution and central tendency in multidimensional spaces.
Real-World Examples of the Spatial Median
The spatial median is used in various real-world applications, such as geographical information systems (GIS), where it helps in finding the central location of a set of geographical points. In finance, the spatial median is used to analyze multivariate financial data, providing a robust measure of central tendency for portfolios and asset returns. In healthcare, the spatial median helps in analyzing multidimensional patient data, identifying central trends and anomalies. Understanding these practical applications can provide valuable context for students, helping them see the relevance of the spatial median in different fields.
Solving Multidimensional Data Problems with the Spatial Median
To effectively solve problems involving multidimensional data, it is crucial to understand the properties and applications of the spatial median. Start by defining the dataset and ensuring it is not concentrated along a single line. Apply the principles of convexity to identify the spatial median, using optimization techniques to minimize the total distance. Consider the computational complexity and use appropriate software tools to simplify calculations. By following these steps, students can solve homework involving the spatial median with confidence and accuracy, applying this knowledge to various statistical analyses.
Conclusion
The spatial median is a powerful concept in multivariate statistics, providing a robust measure of central tendency that is less sensitive to outliers and skewed distributions. By understanding its definition, properties, and applications, students can effectively tackle homework involving the spatial median and apply this knowledge to various fields, such as finance, healthcare, and geographical analysis. Utilizing the principles of convexity and optimization techniques, students can find the spatial median in multidimensional datasets, gaining valuable insights and solving complex statistical problems with confidence.