Using SPSS to Simplify and Clarify Complex Data Analysis Tasks
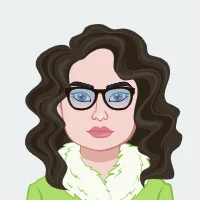
Completing complex statistics assignments can be a daunting task for students, especially when working with intricate datasets and sophisticated analyses. SPSS (Statistical Package for the Social Sciences) emerges as a powerful tool designed to simplify these challenges and provide clarity through its advanced statistical methods. With its user-friendly interface and comprehensive features, SPSS is invaluable for performing a wide range of statistical operations, from basic descriptive statistics to intricate inferential analyses.
The strength of SPSS lies in its ability to manage large datasets efficiently, conduct detailed hypothesis testing, and generate insightful visualizations, such as cross-tabulations and bivariate graphics. This capability allows students to transform complex data into clear, actionable insights, facilitating a more streamlined and accurate analysis process.
Mastering SPSS requires a solid grasp of several critical components: formulating clear and precise research and null hypotheses, developing a robust theoretical framework, conducting comprehensive data analysis, and presenting results according to APA standards. Effective use of SPSS can significantly enhance students' analytical abilities, allowing them to produce high-quality, insightful assignments and achieve a thorough understanding of statistical relationships within their datasets. For those who encounter difficulties with these aspects, seeking assistance to solve your SPSS homework can offer essential support. This guidance helps ensure you manage complex assignments efficiently, confidently interpret your data, and achieve accurate results that reflect a deep grasp of statistical concepts.
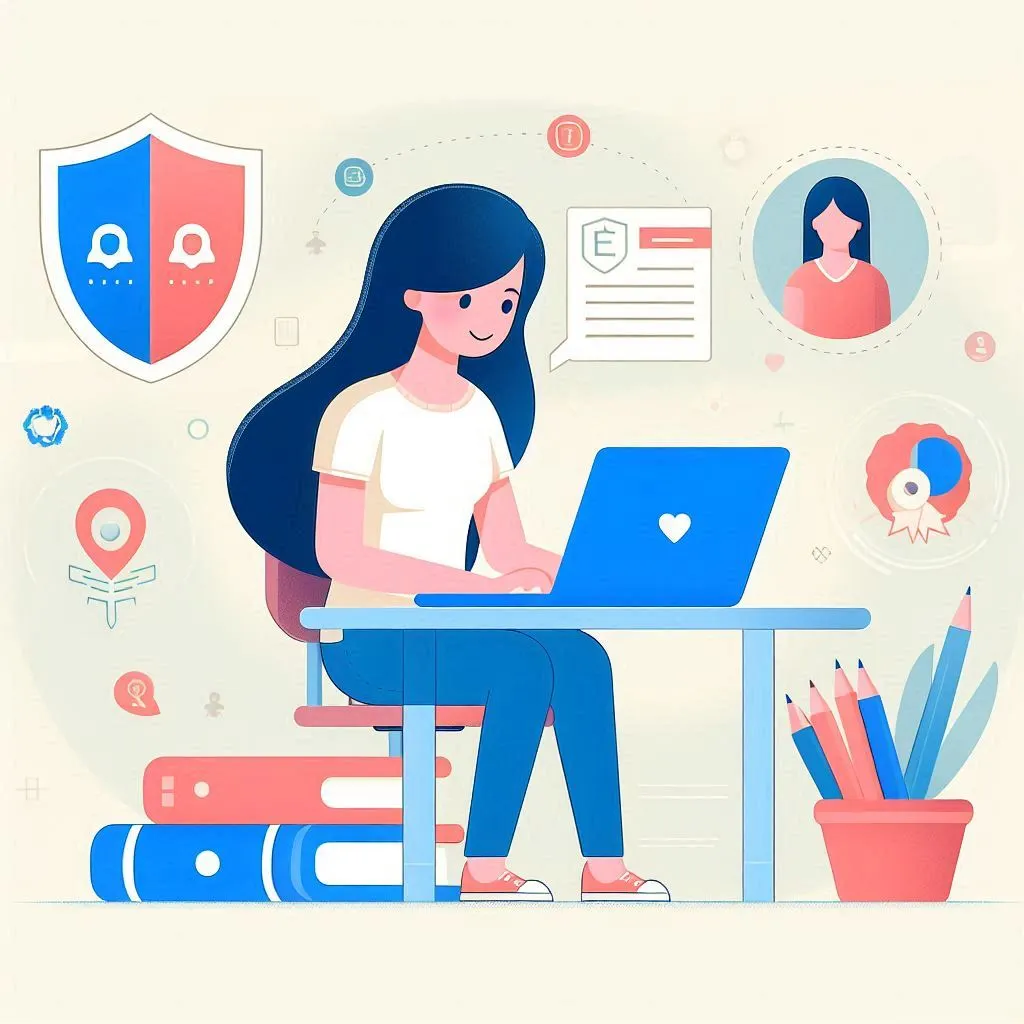
Formulating Hypotheses: A Crucial First Step
Formulating hypotheses is a foundational step in any statistical analysis, guiding the research process and shaping your analysis strategy in SPSS. A hypothesis is a predictive statement about the relationship between variables, serving as the basis for your entire study.
- Research Hypothesis and Null Hypothesis: The research hypothesis (or alternative hypothesis) proposes a specific effect or relationship, such as "students who participate in study groups have higher exam scores compared to those who study alone." This hypothesis directs your investigation, predicting an expected outcome. Conversely, the null hypothesis suggests no effect or relationship, such as "there is no difference in exam scores between students who participate in study groups and those who study alone."
- Crafting Effective Hypotheses: Effective hypotheses should be specific, measurable, and testable. Avoid vague statements; instead, clearly define the variables and their expected relationship. For example, a more precise hypothesis would be, "students using interactive study methods score higher on standardized tests than those using traditional methods." This precision helps in conducting a focused analysis in SPSS and ensures meaningful results.
- Hypotheses and SPSS Analysis: Your hypotheses determine the statistical tests you use in SPSS. For example, to compare means between two groups, you might use a t-test, or for categorical data, a Chi-Square test. Establishing hypotheses before conducting any analysis ensures your approach is systematic and aligned with your research objectives.
A well-formulated hypothesis serves as a roadmap for your research, guiding the selection of statistical tests in SPSS and helping in the accurate interpretation of results.
Developing Your Theory: Understanding the Relationship
Developing a theoretical framework is essential for explaining the expected relationship between variables and guiding your analysis in SPSS. A solid theory provides the rationale for your hypotheses and frames your research.
- Understanding Theoretical Framework: Your theory should offer a plausible explanation for the anticipated relationship between variables. For example, if you hypothesize that "students with higher attendance in lectures achieve better grades," your theory might be based on the idea that regular attendance enhances comprehension and retention of course material. This theory should be informed by existing literature and previous research.
- Constructing a Plausible Theory: To develop a robust theory, integrate relevant concepts and empirical evidence. Review existing research to identify established patterns and theories. For instance, if previous studies show that increased class participation correlates with better academic performance, this supports your theory. Your theory should be specific enough to be testable, logically connecting your independent and dependent variables.
- Theory’s Role in SPSS Analysis: Your theory influences your SPSS analysis design. It helps in formulating precise hypotheses and selecting appropriate statistical tests. If your theory suggests that a demographic factor affects job satisfaction, SPSS can test this hypothesis by comparing groups or examining correlations. A well-developed theory also aids in interpreting results within the context of your theoretical framework.
By thoroughly developing your theory, you lay the groundwork for a focused statistical analysis. This theoretical basis ensures that your hypotheses are well-supported and guides the use of SPSS tools to test and interpret your research questions effectively. With a solid theoretical framework, you are better equipped to complete your Statistics homework, as it provides a clear direction for your analysis and helps in making accurate interpretations of the data.
Creating Cross-Tabulations in SPSS: A Detailed Guide
Creating cross-tabulations in SPSS allows you to explore the relationship between two categorical variables through a contingency table. This step is crucial for examining associations and distributions.
- Steps to Create Cross-Tabulations: Start by opening SPSS and selecting Analyze > Descriptive Statistics > Crosstabs. Place your independent variable (IV) in the Columns box and your dependent variable (DV) in the Rows box. This setup generates a contingency table showing the frequency distribution across variable categories.
- Including Relevant Statistics: For a comprehensive analysis, include counts, row percentages, and Chi-Square test results. For example, your table may display the number of respondents in each category along with their percentages. Chi-Square tests assess the statistical significance of the association between variables. Ensure that the table adheres to APA guidelines with clear titles, sources, and footnotes explaining statistical measures.
- Interpreting Cross-Tabulations: Examine the table to identify patterns or associations between categories. For example, if higher education levels correlate with increased job satisfaction, this pattern should be evident. The Chi-Square test results will indicate if the association is statistically significant.
Cross-tabulations in SPSS provide a detailed view of categorical data relationships. This analysis helps in understanding how variables interact and supports the validation of hypotheses through visual representation.
Designing Bivariate Graphics in SPSS: Enhancing Data Visualization
Designing bivariate graphics in SPSS helps in visualizing the relationship between two variables, making data interpretation clearer and more accessible.
- Creating Bar Charts: To create a bar chart, navigate to Graphs > Chart Builder. Select the bar chart option and drag your IV to the x-axis and your DV to the y-axis. A clustered bar chart is effective for comparing categories within each variable. This visualization helps to see how different groups relate to each other.
- Customizing Your Chart: Customize the chart to enhance readability and APA compliance. Add a descriptive title summarizing the chart's content, and include a source for the data. Adjust colors, labels, and legends to improve clarity. Present percentages rather than raw counts for easier comparison.
- Interpreting Bar Charts: Analyze the bar chart to identify trends or differences between categories. For example, if the chart shows that students in study groups have higher exam scores, this supports your hypothesis visually. Discuss these observations in relation to your research findings.
Bivariate graphics in SPSS make complex data relationships more understandable. Effective visualizations can reveal patterns and trends, enhancing the interpretation and presentation of your results.
Analyzing Results: Interpreting Statistical Output
Analyzing results involves interpreting the statistical output from SPSS to draw meaningful conclusions about your hypotheses.
- Interpreting Hypothesis Tests: Focus on key statistics such as Chi-Square values, p-values, and degrees of freedom. A p-value less than 0.05 typically indicates a significant relationship between variables. Report these values and their implications for your hypotheses test . For instance, if the Chi-Square test shows a significant result, you would reject the null hypothesis and conclude that a relationship exists.
- Drawing Conclusions: Decide whether to reject or retain the null hypothesis based on your SPSS results. If the p-value is significant, reject the null hypothesis and interpret the strength and direction of the relationship. For example, if higher educational attainment is associated with better job satisfaction, explain how this supports your hypothesis.
- Contextualizing Results: Place your findings within the broader context of your study. Relate your results to existing research and theoretical expectations. Discuss any unexpected results and consider potential explanations.
Analyzing SPSS results involves interpreting statistical measures and drawing conclusions about your hypotheses. This process ensures that your findings are well-supported and relevant to your research questions.
Ensuring APA Compliance: Formatting Your SPSS Output
Ensuring APA compliance is crucial for presenting SPSS results professionally and adhering to academic standards.
- APA Formatting Guidelines: Follow APA guidelines for tables and charts. Include clear titles, sources, and explanatory footnotes. Ensure tables and charts are properly aligned and formatted for readability. Adhere to APA standards for statistical measures, such as Chi-Square values and p-values.
- Editing and Presentation: Export SPSS output to a text editor or word processor for final formatting. Review tables and charts for APA compliance and make necessary adjustments. This final editing phase ensures that your presentation is professional and meets academic expectations.
- Consistency and Accuracy: Verify the accuracy of statistical measures and the consistency of formatting. Ensure that your report provides a comprehensive and well-organized presentation of your results.
By adhering to APA standards, you present your SPSS results in a clear and professional manner, enhancing the credibility and readability of your work.
Reviewing and Finalizing Your Analysis: Quality Checks
Reviewing and finalizing your analysis ensures accuracy and completeness before submission.
- Reviewing Your Work: Thoroughly review your SPSS output, including tables, charts, and statistical measures. Check that your hypotheses are clearly stated and your analysis is accurate. Ensure that your visualizations and interpretations are coherent and well-organized.
- Final Adjustments: Make any necessary revisions to improve clarity and coherence. This may involve correcting formatting issues or clarifying statistical measures. Ensure that all aspects of your analysis align with your research objectives and theoretical framework.
- Quality Assurance: Perform a final quality check to ensure your report meets academic standards. Verify the accuracy of data analysis and the professionalism of your presentation. This final review ensures that your analysis is robust and ready for submission.
Reviewing and finalizing your analysis is crucial for producing high-quality research. This final step ensures that your SPSS work is accurate, well-presented, and ready for academic evaluation.
Conclusion
Mastering complex statistics assignments with SPSS requires a systematic approach, from formulating precise hypotheses to interpreting results and ensuring APA compliance. By following the steps outlined in this blog, including hypothesis formulation, theory development, data analysis, and effective presentation, you can enhance your statistical analysis and draw meaningful conclusions from your data.
SPSS is a powerful tool for conducting statistical analysis, but its effectiveness relies on a clear understanding of your research objectives and a rigorous approach to data interpretation. By adhering to best practices and ensuring that your results are presented professionally, you can successfully tackle complex statistics assignments and contribute valuable insights to your field of study.