Innovative Data Visualization Techniques for Statistics
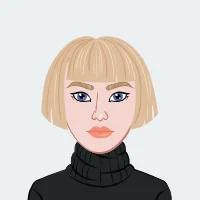
Data visualization is a fundamental practice in statistical analysis, playing a pivotal role in transforming complex datasets into visual representations that enhance comprehension and insight. By employing charts, graphs, and plots, statisticians can effectively communicate patterns, trends, and relationships within data, facilitating informed decision-making and deeper exploration of research findings. This introductory section highlights the critical importance of data visualization in translating intricate numerical data into intuitive visual forms accessible to diverse audiences, including stakeholders in business, academia, and policymaking. Through visual representations, data visualization bridges the gap between raw data and meaningful interpretation, enabling analysts to present findings in a clear, compelling manner that supports evidence-based conclusions and strategic insights. For students seeking assistance in data visualization homework trying these services like "Statistics Homework Help" can be invaluable.
Types of Data Visualization Techniques
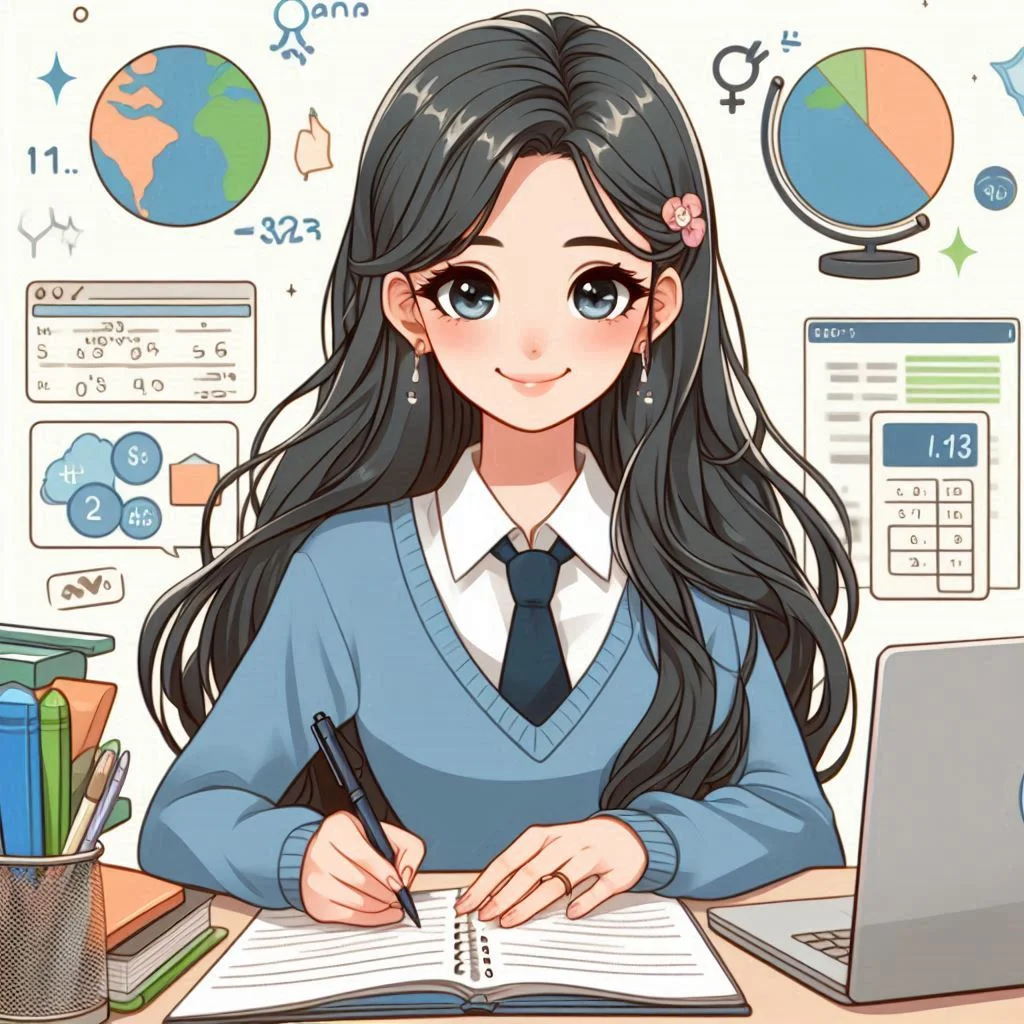
Data visualization techniques encompass a diverse array of methods tailored to different data types and analytical objectives. Each technique serves a unique purpose in uncovering insights and patterns within data:
- Histograms and Frequency Plots: Essential for visualizing data distribution and frequency, histograms present numerical data in bins or intervals, illustrating variability, central tendencies, and outliers critical for statistical analysis. Frequency plots offer a similar perspective, emphasizing data occurrence and distribution across categorical or numerical variables.
- Scatter Plots: Widely used to visualize relationships between two variables, scatter plots plot data points as dots on a graph, facilitating the identification of correlations, clusters, or trends within datasets. They are instrumental in hypothesis testing, pattern recognition, and outlier detection.
- Bar Charts and Pie Charts: Bar charts compare categorical data by representing data values as rectangular bars whose lengths correspond to the values they represent. Pie charts, on the other hand, display proportions within a dataset by dividing a circle into segments, making them effective for illustrating proportions, distributions, and comparisons among categories.
- Heatmaps and Contour Plots: These visualization methods depict data density across two-dimensional spaces. Heatmaps use color gradients to represent the intensity of data values, making patterns and variations visually apparent. Contour plots, meanwhile, use contour lines to map levels of intensity, revealing spatial relationships and trends within multidimensional datasets.
Importance of Data Visualization in Statistical Analysis
Data visualization is indispensable in statistical analysis for its ability to translate complex datasets into visual forms that enhance interpretation and communication. By revealing hidden insights and patterns within data, visualizations facilitate evidence-based decision-making across various sectors, from scientific research and healthcare to business analytics and education. This section underscores how data visualization enables analysts to explore and uncover meaningful trends, correlations, and anomalies not immediately discernible from raw data alone. By presenting data visually, statisticians can convey findings in a compelling and accessible manner, ensuring clarity and facilitating informed decision-making among stakeholders and decision-makers.
Choosing the Right Visualization Technique
Selecting the appropriate visualization technique is crucial for effectively communicating analytical findings and insights. Factors influencing the choice of visualization method include the nature of the data being analyzed, specific research objectives, and the intended audience for the visual representation. This section emphasizes the importance of aligning visualization methods with these factors to ensure clarity and relevance in conveying insights derived from statistical analysis. By choosing the right visualization technique, statisticians can enhance the interpretability of data, highlight key patterns or relationships, and support data-driven conclusions and recommendations tailored to the needs of stakeholders.
Best Practices for Effective Data Visualization
Creating impactful visualizations requires adherence to best practices that optimize clarity, interpretability, and visual appeal. This section outlines essential principles for designing effective visualizations:
- Simplicity and Clarity: Simplify visualizations to focus on the most relevant insights and avoid unnecessary complexity that may obscure the message.
- Color and Design Principles: Utilize appropriate color schemes and design elements to enhance readability and highlight important data points or trends within the visualization. Effective use of color can draw attention to key insights while ensuring that visualizations remain accessible to color-blind viewers.
- Annotations and Labels: Provide clear labels, titles, and annotations to contextualize data points and facilitate understanding among viewers. Annotations help explain trends or anomalies in the data, guiding the audience through the visualization and reinforcing key messages.
- Consistency and Accuracy: Maintain visual consistency and ensure accuracy in representing data to prevent misinterpretation and support reliable data analysis and decision-making. Consistent use of scales, axes, and data units across different visualizations helps maintain coherence and comparability within a dataset.
Tools and Software for Data Visualization
Effective data visualization is paramount in statistical analysis, enabling researchers to distill complex datasets into actionable insights. Various tools and software platforms offer robust capabilities tailored to diverse analytical needs:
Data visualization tools empower statisticians and analysts to transform complex datasets into actionable insights. These platforms provide versatile solutions for exploratory data analysis, presentation-ready graphics, and interactive visualizations across diverse research domains.
- R: Renowned for its extensive collection of packages, including ggplot2 and plotly, R provides robust tools for generating customizable and publication-quality visualizations across diverse datasets and research domains. Its flexibility allows statisticians to create complex visualizations tailored to specific analytical needs, from exploratory data analysis to presentation-ready graphics.
- Python: Supported by libraries such as Matplotlib, Seaborn, and Plotly, Python facilitates the creation of sophisticated statistical plots and interactive visualizations. Python's versatility and integration with data science libraries make it a popular choice for data visualization tasks, enabling analysts to develop interactive dashboards, geographical maps, and trend analyses with ease.
- Tableau and Power BI: These platforms specialize in creating interactive dashboards and visual analytics solutions, empowering users to explore data dynamically and derive actionable insights for decision support and business intelligence applications. Tableau's drag-and-drop interface and robust data connectivity options make it accessible for users at various skill levels, while Power BI's integration with Microsoft products enables seamless data visualization and reporting within enterprise environments.
Case Studies and Examples
Explore how data visualization techniques have made a significant impact across various domains:
Data visualization techniques are pivotal in transforming complex data into actionable insights across diverse domains. These visualizations play a crucial role in understanding trends, patterns, and relationships within datasets, empowering decision-makers to derive meaningful conclusions and drive strategic initiatives.
- Healthcare: Visualizing patient data plays a crucial role in identifying disease trends, monitoring treatment outcomes, and improving healthcare delivery. Hospitals leverage visual analytics to track patient demographics, treatment effectiveness, and resource allocation, guiding healthcare policies and enhancing patient care quality. For example, interactive dashboards visualize hospital performance metrics, patient outcomes, and treatment protocols, enabling healthcare providers to optimize clinical workflows and allocate resources effectively in response to evolving healthcare challenges.
- Finance: Data visualization is instrumental in analyzing market trends, portfolio performance, and economic indicators for informed decision-making in financial institutions. Financial analysts use interactive visual analytics to monitor stock market fluctuations, predict investment trends, and optimize portfolio strategies.
- Education: Visualizations enable educational institutions to analyze student performance data, track learning outcomes, and inform evidence-based educational policies. Data dashboards visualize student achievement metrics, attendance patterns, and learning progress across academic programs.
Conclusion
In conclusion, data visualization techniques are essential for statisticians and analysts to effectively communicate complex data insights. By leveraging diverse methods tailored to data characteristics and research goals, professionals can enhance decision-making processes, drive innovation, and contribute to evidence-based advancements across industries. Mastery of data visualization principles and tools equips analysts to extract meaningful insights, communicate findings clearly, and navigate the complexities of modern data analytics effectively. As data volumes grow, proficiency in visualization remains a critical skill for unlocking actionable insights and facilitating informed decisions that drive organizational success and societal progress.
By embracing best practices, leveraging advanced tools, and harnessing the storytelling power of visualizations, statisticians can effectively communicate the narrative within their data, empower stakeholders with actionable insights, and pave the way for informed decisions that drive organizational success and societal advancement.