Understanding the Dynamics of Customer Preferences with Key Driver Analysis
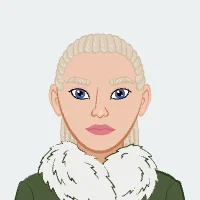
Statistics homework involving key driver analysis can be particularly challenging, especially when working with complex datasets and multiple variables. These tasks require not only a deep understanding of the data but also the ability to identify the factors that most significantly influence outcomes, such as customer behavior. Understanding these key drivers is essential, as they directly impact strategic decisions related to brand preference, customer satisfaction, and purchase intent. For both students and professionals, mastering key driver analysis is more than just a technical exercise; it involves deriving meaningful insights from data that can inform critical business decisions.
This blog outlines a structured approach to effectively tackle such homework, offering the methodologies and techniques necessary to analyze customer experience data with precision. By carefully navigating the intricacies of your dataset and applying advanced statistical methods, you can develop the expertise needed to perform a comprehensive key driver analysis. Whether you need to solve your statistics homework or handle a more complex project, following these steps will not only deepen your understanding of the statistical processes involved but also empower you to confidently apply these methods to any homework requiring a thorough examination of customer experience, regardless of the specific dataset or variables involved. Whether your focus is on identifying the drivers of customer loyalty, uncovering the essential elements of brand perception, or assessing the impact of marketing strategies on purchasing behavior, this article will provide you with the essential insights to handle even the most complex homework with professionalism and accuracy.
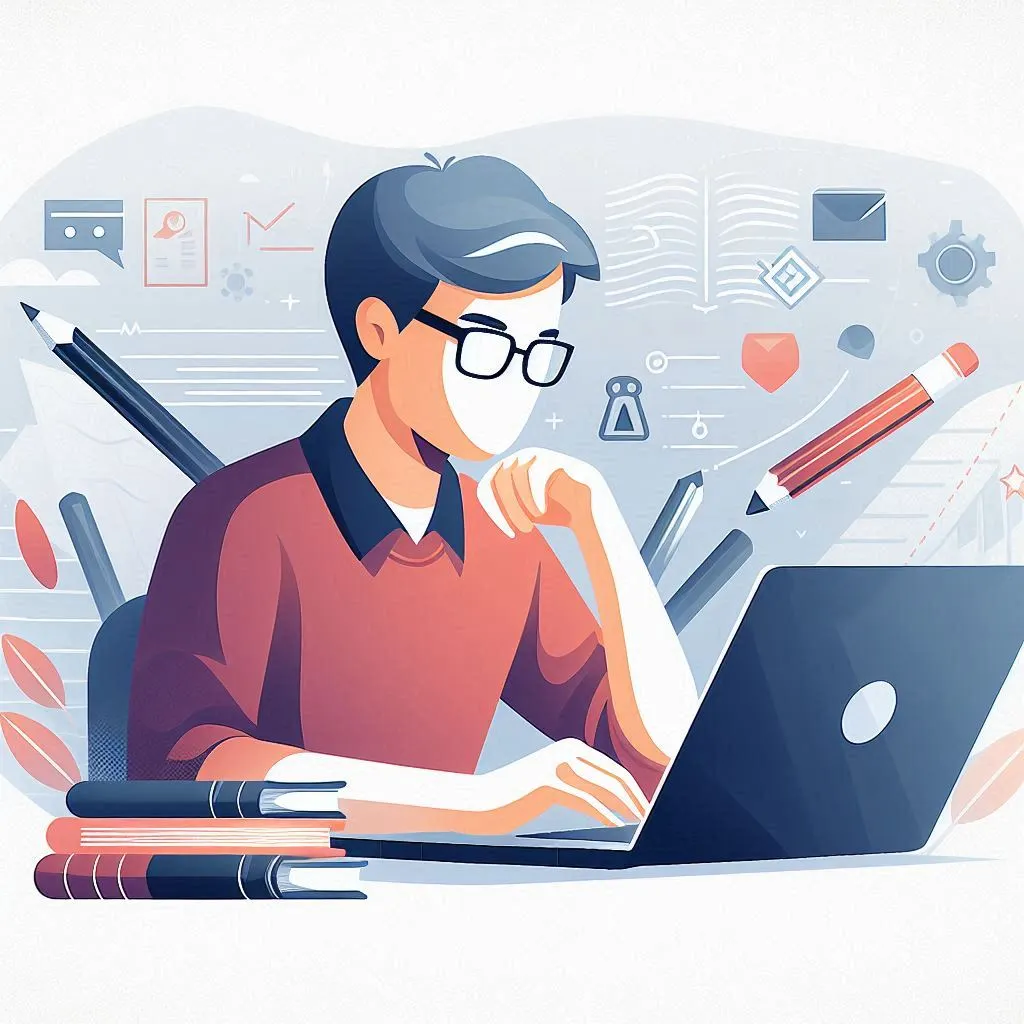
Understanding the Dataset and Key Variables
The first and most critical step in any statistical analysis is to gain a thorough understanding of the dataset you're working with. This involves not only familiarizing yourself with the variables but also comprehending the context in which the data was collected. For example, in the case of the grocery dataset provided in the homework, the various performance attributes related to the client’s brand serve as the predictors, while the outcomes of interest are "Preference" and "Purchase Intent." Each of these predictors, such as product quality (Perf_products), brand perception (Perf_brands), and ease of shopping (Perf_shopeasy), represent different aspects of the customer’s experience with the brand. Understanding these attributes is essential for interpreting the analysis results accurately.
To effectively understand your dataset, start by exploring the variables and their labels. Use tools like SPSS or Excel to inspect the data, examine the distributions of the variables, and identify any potential outliers or missing values. It's also important to consider the scale of measurement for each variable, as this will impact how they are used in the analysis. For instance, categorical variables may need to be dummy-coded before they can be included in a linear regression model. Additionally, understanding the full context of each variable, including how they were measured and what they represent in the real world, will allow you to interpret the results more meaningfully.
In the context of this homework, it is particularly important to pay attention to how the performance attributes relate to the overall customer experience. For instance, attributes like "Perf_shopeasy" may directly influence the ease with which customers can navigate the store, which in turn affects their overall brand preference. Similarly, "Perf_products" may reflect the quality of the products offered, which could be a significant factor in driving purchase intent. By thoroughly understanding these variables, you lay the groundwork for a successful analysis that accurately reflects the factors driving customer behavior.
Setting Up the Analysis: Linear Regression Basics
Once you have a solid understanding of the dataset and the variables, the next step is to set up your analysis using linear regression. Linear regression is one of the most widely used statistical methods for determining the relationship between a dependent variable (the outcome) and one or more independent variables (the predictors). In key driver analysis, linear regression is used to identify which predictors are the most significant drivers of the outcome, be it customer preference or purchase intent.
Setting up a linear regression analysis involves several critical steps. Initially, it is essential to select the appropriate statistical software for your analysis. Tools such as SPSS, R, and Excel are commonly utilized for linear regression, each providing distinct advantages. Once the software is chosen, the next step is to input your outcome variable (e.g., Preference) along with your predictor variables (e.g., Perf_products, Perf_brands, Perf_organic). To ensure that you complete your linear regression homework with precision, it is vital to correctly specify your model. This includes incorporating all relevant predictors and avoiding the omission of any key variables that may impact the outcome. Proper model specification is crucial for generating accurate and insightful results.
Before running the analysis, it's essential to check that the assumptions of linear regression are met. These include linearity (the relationship between the predictors and the outcome is linear), independence (the observations are independent of one another), and homoscedasticity (the variance of the errors is constant across all levels of the predictors). Violating these assumptions can lead to biased or misleading results, so it’s important to conduct diagnostic tests, such as plotting residuals or using variance inflation factors (VIFs), to ensure that your model is appropriate.
Running the analysis involves fitting the linear regression model to your data and generating output that includes various statistics, such as the R-squared value, p-values for each predictor, and standardized beta coefficients. These statistics provide information about the overall fit of the model, the significance of each predictor, and the strength and direction of the relationships between the predictors and the outcome. For example, a high R-squared value indicates that a large proportion of the variance in the outcome is explained by the predictors, while a low p-value suggests that a predictor is statistically significant.
Running the Key Driver Analysis: Identifying Key Attributes
With your linear regression model set up, the next step is to run the key driver analysis to identify which attributes are the most significant drivers of the outcome. This is the heart of the analysis, where you determine the relative importance of each predictor and gain insights into which factors most strongly influence customer behavior. The goal is to identify the key drivers – the predictors that have the greatest impact on the outcome, whether it’s customer preference or purchase intent.
Running the key driver analysis involves executing the linear regression in your chosen statistical software and carefully examining the output. Start by looking at the model fit statistics, such as the R-squared value and the F-statistic. These statistics tell you how well the model explains the variance in the outcome and whether the overall model is statistically significant. A high R-squared value indicates that the predictors collectively explain a large portion of the variance in the outcome, while a significant F-statistic suggests that the model is a good fit for the data.
Next, examine the standardized beta coefficients for each predictor. These coefficients indicate the strength and direction of the relationship between each predictor and the outcome. A positive beta coefficient means that as the predictor increases, the outcome also increases, while a negative beta coefficient indicates the opposite. The size of the beta coefficient reflects the relative importance of the predictor – larger coefficients indicate stronger relationships. It’s also important to consider the statistical significance of each coefficient, typically assessed using p-values. Predictors with low p-values (usually less than 0.05) are considered statistically significant, meaning that their relationship with the outcome is unlikely to be due to chance.
After identifying the key drivers, the next step is to rank them in order of importance based on their standardized beta coefficients and p-values. This ranking helps you prioritize which attributes are the most critical to focus on in terms of influencing customer behavior. For example, if "Perf_shopeasy" has a large positive beta coefficient and a significant p-value, it suggests that ease of shopping is a major driver of customer preference. On the other hand, if "Perf_bake" has a small or non-significant beta coefficient, it may be less important in driving customer behavior.
Interpreting the Results: Deriving Insights
Interpreting the results of your key driver analysis is a critical step that transforms raw statistical output into actionable business insights. This involves not just understanding which predictors are significant, but also thinking about what these results mean for the client or business. The goal is to derive insights that can guide strategic decision-making, whether it’s improving customer experience, enhancing brand loyalty, or driving sales.
Start by focusing on the key drivers identified in your analysis. Consider how each significant predictor influences the outcome and what this means for the client’s brand strategy. For instance, if "Perf_products" is a significant driver of both preference and purchase intent, it suggests that product quality is a crucial factor for the brand. This insight might lead to recommendations for the client to invest in product development or quality control to enhance customer satisfaction and loyalty.
It’s also important to consider the broader implications of your findings. For example, if "Perf_shopeasy" is a key driver of customer preference, it suggests that the ease of shopping experience plays a critical role in shaping customers’ perceptions of the brand. This might prompt the client to focus on optimizing store layouts, streamlining the checkout process, or improving online shopping interfaces. Similarly, if "Perf_bakequal" (bakery quality) is a significant driver of purchase intent, it indicates that the bakery section is an important area for the client to focus on to increase sales.
Another important aspect of interpretation is understanding the relationships between the predictors. In some cases, predictors may be correlated with one another, which can complicate the interpretation of the results. For instance, if "Perf_organic" (preference for organic products) and "Perf_products" (overall product performance) are both significant drivers, it’s important to consider how these factors interact. Perhaps customers who value organic products also have higher overall expectations for product quality, which could influence their brand preference and purchase decisions.
Finally, it’s essential to think about the practical applications of your findings. How can the client use this information to improve their business? What specific actions should they take based on the key drivers identified in the analysis? For example, if "Perf_coupons" is a significant driver of purchase intent, the client might consider implementing more targeted coupon campaigns to encourage repeat purchases. The insights you derive from the analysis should be actionable and directly tied to improving the client’s business outcomes.
Comparing Multiple Analyses: Cross-Analysis Insights
In many homework, particularly those involving customer experience data, you may be required to run multiple key driver analyses and compare the results. This cross-analysis allows you to gain a more holistic understanding of the factors influencing different aspects of customer behavior. In the context of the given homework, you would compare the results of the key driver analysis for brand preference with those for purchase intent, providing insights into the overall customer experience.
To begin the comparison, start by reviewing the key drivers identified in each analysis. Are the same attributes significant in both cases, or do different factors drive preference versus purchase intent? For example, you might find that product quality ("Perf_products") is a key driver of both preference and purchase intent, indicating its overall importance in the customer experience. Alternatively, you might find that certain attributes, such as "Perf_shopeasy," are more important for preference, while others, like "Perf_coupons," are more critical for driving purchase intent.
Next, consider how the relative importance of the key drivers compares across the analyses. For instance, if "Perf_organic" is a significant driver of preference but not purchase intent, it suggests that while customers who prefer organic products are more likely to choose the brand, this preference may not necessarily translate into actual purchases. This insight could inform strategies to convert brand preference into sales, such as offering organic products at competitive prices or highlighting their benefits more effectively in marketing campaigns.
Finally, use the cross-analysis to derive broader strategic insights. By comparing the key drivers across different outcomes, you can identify overarching themes and patterns that are consistent across the customer experience. For example, if ease of shopping ("Perf_shopeasy") consistently emerges as a key driver across multiple analyses, it suggests that improving the shopping experience should be a top priority for the client. Similarly, if certain attributes, like "Perf_bakequal" (bakery quality), are only significant in specific analyses, it indicates areas where the client can make targeted improvements to enhance customer satisfaction and drive specific business outcomes.
Conclusion
Key driver analysis is a powerful tool for uncovering the factors that drive customer behavior, making it an essential skill for anyone involved in statistics homework. By following the step-by-step approach outlined in this guide – from understanding the dataset and setting up the analysis to running the regression and interpreting the results – you can confidently tackle even the most complex homework. Whether you're analyzing customer experience data for brand preference, purchase intent, or other outcomes, mastering key driver analysis will enable you to derive actionable insights that can guide strategic decision-making and drive business success.