Exploratory Factor Analysis using SPSS: Unveiling Insights from Statistical Data
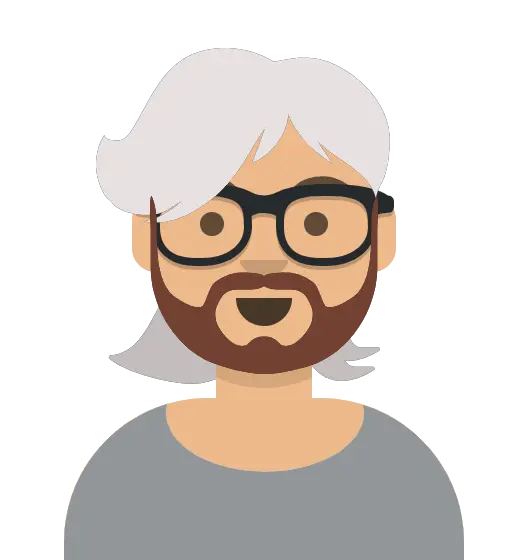
- Exploratory Factor Analysis (EFA) is a powerful statistical technique for identifying latent variables and relationships in a dataset. It enables researchers to investigate and comprehend the underlying structure of their data in the absence of predefined hypotheses. EFA seeks to identify and extract latent factors that explain data variation by examining the interrelationships between observed variables. This technique has grown in popularity across a wide range of disciplines, including psychology, sociology, marketing, and finance, because it allows researchers to gain meaningful insights and a deeper understanding of complex phenomena.
- In the world of research and data analysis, determining the underlying structure and dimensions of a dataset can be difficult. Variables may be related, but it is not always clear how they are related or what latent factors they represent. This is where exploratory factor analysis can help. Researchers can use EFA to uncover hidden patterns and structures that are not visible in raw data, allowing them to identify the underlying factors that contribute to the observed variations.
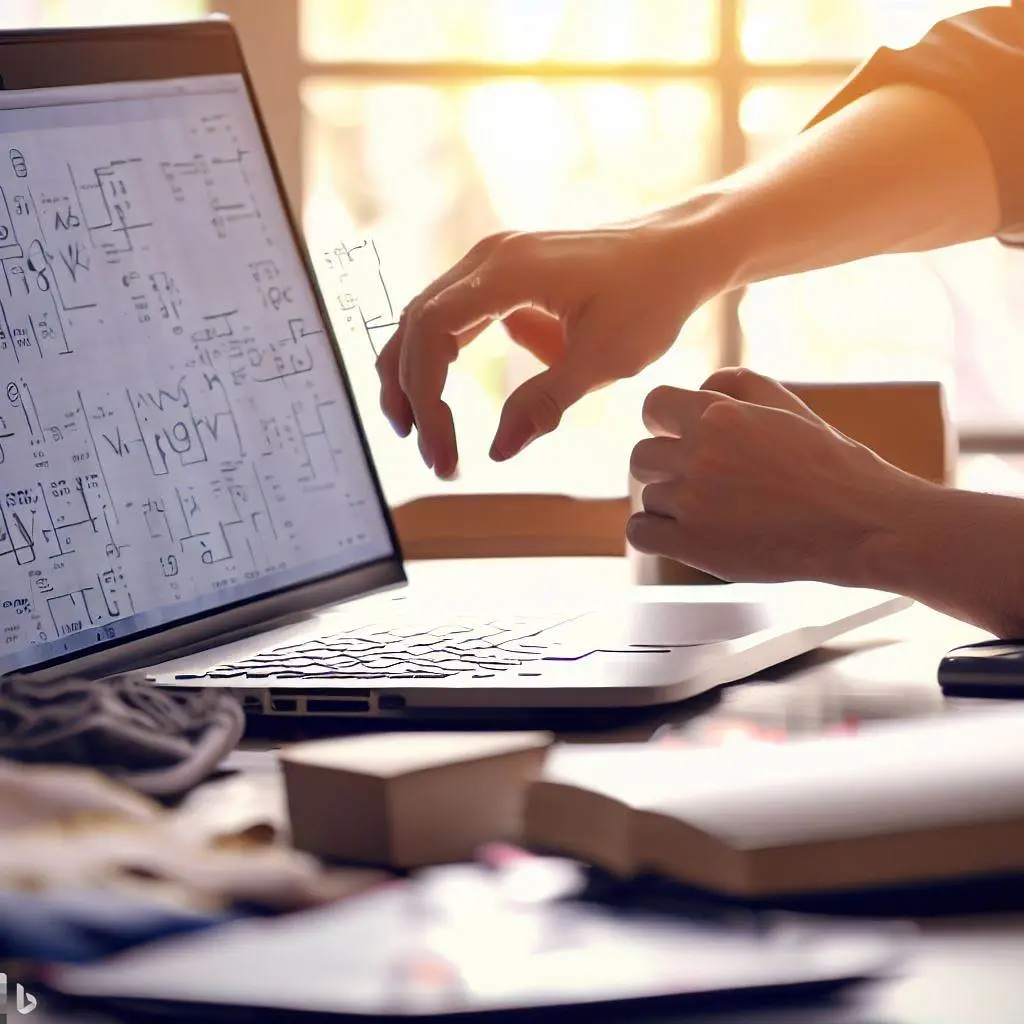
- There are several key steps involved in conducting exploratory factor analysis. To begin, researchers must prepare their data by dealing with missing values, outliers, and determining sample size adequacy. Following that, factor extraction techniques are used to identify the latent factors in the dataset. These techniques, which include Principal Component Analysis (PCA), Principal Axis Factoring (PAF), and Maximum Likelihood (ML) estimation, estimate the underlying factors based on the intercorrelations of the observed variables.
- After extracting the factors, researchers rotate them to produce a more interpretable and meaningful factor solution. Orthogonal rotations, such as Varimax or Quartimax, produce uncorrelated factors, whereas oblique rotations, such as Promax, allow for factor correlation. Factor interpretation is an important step that involves examining factor loadings as well as considering the conceptual meaning of the variables in order to assign meaningful labels to the factors.
- EFA is aided by statistical software such as the Statistical Package for the Social Sciences (SPSS), which provides user-friendly interfaces and analysis tools. SPSS simplifies the process and enables researchers with varying statistical expertise to conduct effective exploratory factor analysis.
- Researchers can gain insights into the underlying structure of their data, identify the key factors driving observed variations, and potentially discover new knowledge or relationships by using EFA. This data can be used to inform decision-making processes, improve measurement instruments, and guide future research in the field.
- We will provide a comprehensive guide to exploratory factor analysis using SPSS in this blog. We will look at the theoretical foundations of EFA, the step-by-step process of conducting the analysis, and its applications and limitations. By the end of this blog, you will have a firm grasp on how to apply EFA in your own research and harness the power of statistical analysis to elicit meaningful insights from your data.
Exploratory Factor Analysis Basics:
When researchers do not have a specific hypothesis about the underlying structure of a dataset, they use exploratory factor analysis. Instead, EFA enables an exploratory examination of the data in order to identify latent factors driving the observed variation. These are unobservable variables that can explain a set of measured variables' common variance.
The purpose of exploratory factor analysis is to answer two major questions: (1) How many variables are there in the data? (2) How are the variables related to each factor? EFA provides a deeper understanding of the underlying structure and dimensionality of the data by answering these questions.
Exploratory Factor Analysis Theoretical Foundation:
Before delving into the practical application of EFA with SPSS, it is critical to understand the theoretical underpinnings of this statistical technique. EFA is based on factor analysis, a statistical method developed in the early twentieth century by Charles Spearman. Spearman's work popularized the concept of general intelligence, implying that differences in cognitive ability could be explained by a single underlying factor. This concept paved the way for the more widespread use of factor analysis in a variety of disciplines.
The assumption of factor analysis is that observed variables are influenced by a smaller number of latent factors. These latent factors capture the observed variables' shared variance. EFA seeks to identify these latent factors and comprehend their relationship to the observed variables.
Exploratory Factor Analysis Steps:
EFA is a systematic series of steps that helps reveal the underlying factor structure in a dataset. The following sections outline the key steps in conducting exploratory factor analysis with SPSS:
Preparation of Data:
It is critical to ensure that the dataset is properly prepared before beginning the analysis. This includes dealing with missing data, looking for outliers, and ensuring that the sample size is adequate. SPSS includes a number of tools to help with data cleaning and preparation.
Extraction of Factors:
The process of extracting the underlying factors from a dataset is known as factor extraction. Several methods, such as Principal Component Analysis (PCA), Principal Axis Factoring (PAF), and Maximum Likelihood (ML) estimation, can be used. Each method has its own assumptions and considerations that must be taken into account based on the data characteristics.
Principal Component Analysis (PCA) is a popular method for accounting for the greatest amount of variance in data. It generates principal components, which are linear combinations of the observed variables that are ranked by the amount of variance they explain. Principal Axis Factoring (PAF) and Maximum Likelihood (ML) estimation, on the other hand, are concerned with estimating the common factors underlying the observed variables.
Rotation of Factors:
The goal of factor rotation is to produce a more interpretable and meaningful factor solution. The two main types of rotation techniques are orthogonal and oblique rotations. Orthogonal rotation results in uncorrelated factors, whereas oblique rotation allows for factor correlation. Varimax, Quartimax, and Equamax are common orthogonal rotation methods, while Promax and Oblimin are common oblique rotation methods.
The rotation method used is determined by the nature of the data and the research objectives. The factor loadings after rotation are frequently examined by researchers in order to interpret the factors and their relationships with the observed variables.
Interpretation of Factors:
The interpretation of the EFA factors is a critical step. The factor loadings, which indicate the strength and direction of the relationship between each variable and the underlying factor, must be examined by researchers. Variables with higher factor loadings on a specific factor have a stronger relationship with that factor.
Consideration of the conceptual meaning of the variables, in addition to factor loadings, can aid in interpreting the factors and assigning meaningful labels to them. To determine the number of factors to retain, researchers may also consider the eigenvalues, scree plot, and cumulative variance explained.
Evaluation of Reliability and Validity:
The assessment of reliability and validity is critical to ensuring the robustness of the factor solution. Cronbach's alpha, factor congruence, and construct validity are all measures that can be used to assess the reliability and validity of the factors identified through EFA.
Cronbach's alpha measures the internal consistency of each factor's items, indicating how well they measure the same underlying construct. Factor congruence investigates the similarity of factor loadings across samples or subgroups. Construct validity involves determining how well the factors match theoretical expectations and previous research.
Using SPSS to Conduct Exploratory Factor Analysis:
SPSS is a popular statistical software that has an easy-to-use interface for conducting exploratory factor analysis. The software includes a comprehensive set of tools and options to help with each step of the analysis. SPSS simplifies the EFA process, from data preparation to factor extraction, rotation, and interpretation, making it accessible to researchers with varying statistical expertise.
SPSS includes a number of menus and dialog boxes that are specifically designed for factor analysis. Users can define variables, select factor extraction and rotation methods, and obtain factor loadings, communalities, and other relevant statistics using these tools. SPSS also provides graphical outputs to aid in the interpretation of the results, such as scree plots and factor correlation matrices.
Exploratory Factor Analysis Applications and Limitations:
Exploratory Factor Analysis has numerous applications in fields such as psychology, sociology, marketing, and finance. It allows scientists to identify latent variables that can explain complex phenomena. EFA can be used in psychology to uncover underlying dimensions of personality traits or psychological constructs. EFA can aid in the identification of factors influencing consumer preferences and behavior in marketing.
However, it is critical to recognize EFA's limitations. EFA results can be sample-dependent, which means that different samples may produce different factor solutions. Factor interpretation is subjective and necessitates careful consideration of the context and theoretical foundation. Furthermore, EFA is an exploratory technique that does not provide confirmatory evidence for the factors that have been identified. Confirmatory Factor Analysis (CFA) is frequently used as a step after factor structure validation.
Conclusion:
Exploratory Factor Analysis with SPSS is a useful statistical technique for determining the underlying factor structure in a dataset. Researchers can gain a better understanding of the relationships between observed variables by identifying latent variables. This blog has provided a comprehensive overview of the theoretical foundations of EFA, the steps involved in conducting the analysis, and the practical application of SPSS for EFA. With this information, you can use EFA to extract meaningful insights from your data and advance your research efforts.