Using ANOVA and GIS Together to Analyze and Visualize Spatial Data
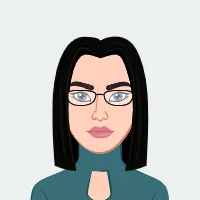
Statistics and Geographic Information Systems (GIS) are essential tools for analyzing intricate datasets, especially in academic environments. Each field offers distinct methodologies for data analysis, but their combined application provides a powerful means for gaining profound insights. Statistics, through its various analytical techniques, enables thorough examination of data patterns, relationships, and variability. GIS, conversely, facilitates the visualization and spatial analysis of data, adding a geographical perspective that uncovers trends and relationships that might otherwise be obscured.
Integrating these disciplines offers a robust framework for data analysis and interpretation. For instance, incorporating ANOVA (Analysis of Variance) with GIS allows you to execute detailed spatial analyses while simultaneously assessing statistical significance. This synergy is particularly advantageous in projects involving geographic data, where understanding how different variables interact across spatial dimensions is crucial.
We will delve into the integration of ANOVA with GIS for homework assignments, using the Mexican Border Project as a case study. This project encompasses the analysis of demographic and geographic data concerning border areas and provides a practical context for applying these techniques. By combining GIS and ANOVA, you can achieve a detailed analysis that not only identifies statistical differences but also visualizes these differences spatially, offering a deeper comprehension of the data. If you’re looking to solve your ANOVA homework efficiently, mastering this integration will enhance your ability to perform nuanced analyses and present your findings with clarity.
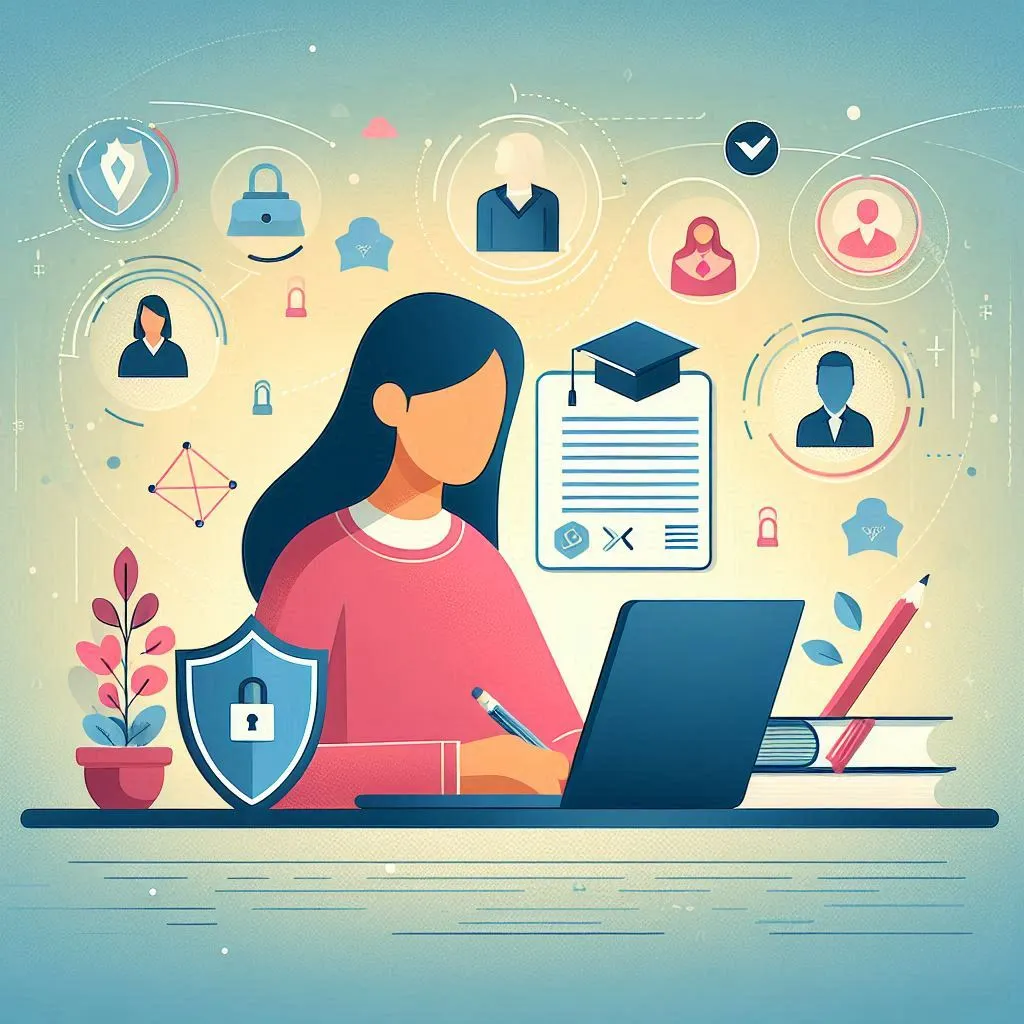
By exploring these methods, you will gain valuable insights into how to apply ANOVA within GIS frameworks, allowing you to conduct sophisticated spatial analyses and generate meaningful conclusions from your data. This guide will equip you with the skills needed to tackle complex homework assignments and prepare for advanced research, ensuring you can effectively merge statistical and spatial data for comprehensive analyses.
Understanding Homework Requirements
Effective analysis starts with a clear understanding of your homework requirements. Whether you're tackling a project like the Mexican Border Project or any other GIS assignment, a comprehensive grasp of the task is essential for achieving meaningful results.
Key Elements to Focus On:
- Detailed Review: Begin by meticulously reading the assignment instructions. This involves not only understanding the project’s objectives but also the specific type of analysis required and the expected deliverables. For the Mexican Border Project, this could mean understanding how to analyze demographic shifts in relation to border policies.
- Objective Clarity: Ensure that you clearly define the goals for each component of your assignment. For instance, if the project requires you to analyze demographic changes within buffer zones using ANOVA, understand how this analysis will support the broader objectives of the project.
- Contextual Understanding: Acquire a comprehensive understanding of the broader context of your assignment. For the Mexican Border Project, this involves knowing the historical, political, and socio-economic factors that might affect the data you are analyzing.
Effective Strategies for Understanding Requirements:
- Break Down the Assignment: Dissect the project into smaller, manageable tasks such as data collection, GIS mapping, and statistical analysis. This approach not only organizes your workflow but ensures that each part of the assignment is completed with attention to detail.
- Create a Detailed Checklist: Develop a checklist based on the assignment instructions to systematically track your progress. This helps in ensuring that all aspects of the homework are addressed thoroughly and in a structured manner.
By thoroughly understanding the homework requirements, you lay a solid foundation for successfully integrating GIS and statistical techniques like ANOVA, setting the stage for effective and insightful analysis.
Collecting and Preparing Data for GIS Analysis: Building a Reliable Foundation
Data collection and preparation are pivotal in GIS analysis, especially when paired with statistical methods like ANOVA. For complex projects such as the Mexican Border Project, ensuring the accuracy and organization of your data is crucial.
Best Practices for Data Collection:
- Source Reliability: Opt for data from reliable sources such as governmental databases, reputable academic research, or recognized organizations. Reliable data is critical for ensuring the validity of your analysis and the credibility of your results.
- Data Relevance: Gather data that is directly relevant to the objectives of your assignment. For example, if your analysis involves population density, ensure that you collect current and specific demographic data relevant to the buffer zones around the border.
- Understanding Metadata: Metadata provides crucial information about your data, including its source, accuracy, and format. Reviewing metadata is essential for assessing the quality of your data and its appropriateness for your analysis.
Preparing Data for ANOVA Analysis:
- Data Cleaning: Thoroughly clean your data to eliminate errors, inconsistencies, or outliers. This step ensures that your analysis is based on accurate and reliable information, which is essential for obtaining valid results.
- Data Integration: Align and format your GIS data layers and demographic data to prepare them for ANOVA. Ensure that the data formats are compatible, such as converting shapefiles and CSV files into a unified format for analysis.
Example Preparation: For the Mexican Border Project, make sure that buffer zones are accurately delineated and that demographic data within these zones is cleaned and formatted properly for subsequent statistical analysis.
Integrating Data Layers and Conducting ANOVA: Combining Spatial and Statistical Analysis
With your data collected and prepared, the next step is to integrate it into GIS and apply ANOVA to analyze spatial patterns and variations. This process involves several critical steps to ensure that your analysis is accurate and insightful. First, you need to carefully import your datasets into the GIS platform, making sure they are correctly aligned with the base map and coordinate system. Proper alignment is crucial for accurate spatial analysis and visualization.
Once your data layers are correctly integrated, you can proceed to apply ANOVA, a powerful statistical technique used to determine if there are statistically significant differences between group means. In the context of GIS, ANOVA can help you analyze variations in your spatial data across different regions or categories. For instance, if you're examining demographic data within different buffer zones along the Mexican border, ANOVA can reveal whether there are significant differences in population density, economic indicators, or other variables between these zones. By mastering these techniques, you can effectively complete your Statistics homework and ensure that your findings are both statistically sound and spatially relevant.
Georeferencing and Coordinate Systems:
- Georeferencing: Ensure that all data layers are aligned to a common spatial reference system to enable accurate overlay. Misalignment can result in errors in spatial analysis and interpretation.
- Coordinate System Alignment: Standardize the coordinate systems of all data layers to maintain consistency across your analysis. Different datasets may use various coordinate systems, so aligning them is crucial for accurate spatial representation.
Buffer Analysis and ANOVA:
- Buffer Zones: Create buffer zones around key geographic features, such as the US-Mexican border, to categorize data for ANOVA. For example, you might analyze demographic variables within different buffer zones to assess differences in population density.
- ANOVA Application: Use ANOVA to test for significant differences in demographic variables across the buffer zones. This involves comparing means to determine if variations are statistically significant and understanding how these differences relate to your spatial data.
Advanced Techniques:
- Overlay Analysis: Combine multiple data layers to explore relationships between different factors. For instance, overlay demographic data with environmental factors to see how they interact and influence each other.
- Intersect and Union Operations: Perform GIS operations like intersect and union to identify overlapping areas between data layers, such as intersections between buffer zones and land use types.
Example Analysis: In the Mexican Border Project, perform ANOVA to examine whether income levels significantly differ across various buffer zones around the border. This analysis helps in understanding how proximity to the border influences socioeconomic variables.
Statistical Analysis within GIS: Enhancing Insights with ANOVA
Combining ANOVA with GIS provides powerful analytical capabilities, allowing you to uncover patterns and trends that might be obscured through basic observation alone.
Hot Spot Analysis and ANOVA:
- Hot Spot Analysis: Identify clusters of high or low values within your data. Integrate this analysis with ANOVA to determine if these clusters correspond to significant variations in demographic or environmental variables.
Regression Analysis and ANOVA:
- Regression Analysis: Use regression models to explore relationships between variables, such as the impact of proximity to the border on crime rates or environmental conditions. Apply ANOVA to test the significance of these relationships and understand the underlying patterns.
Data Normalization and ANOVA:
- Normalization: Normalize your data to facilitate valid comparisons between different areas. For example, adjust population data by area to compare density across different buffer zones and ensure that your ANOVA results are meaningful.
Example Integration: After conducting ANOVA, use Hot Spot Analysis to visualize areas with significant variations in population density within buffer zones. This combined approach provides a comprehensive view of your data and enhances the depth of your analysis.
Customizing Maps for Effective Communication: Presenting ANOVA Results Clearly
Creating effective maps is crucial for communicating the results of your GIS and statistical analysis. Tailoring your maps to reflect ANOVA results helps in presenting complex information in a clear and visually engaging manner.
Effective Use of Color and Texture:
- Color and Texture: Select colors and textures that effectively distinguish between different data categories and buffer zones. Ensure these visual elements enhance the readability of your map and align with your ANOVA findings.
Legend and Labels:
- Legend: Design a detailed legend that explains the symbols and colors used on your map. This helps viewers interpret the spatial distribution and significance of the ANOVA results accurately.
- Labels: Strategically place labels to identify key features and findings without overcrowding the map. Ensure that important data points and results are easily identifiable.
Map Layout and Presentation:
- Layout: Arrange your map elements to highlight the results of your ANOVA analysis. Include titles, scale bars, and north arrows to provide context and improve the map’s clarity.
- Exporting the Map: Export your map in a high-resolution format to maintain quality. Ensure that the final product is polished, professional, and suitable for presentation or submission.
Example Customization: After performing ANOVA, create a map displaying different colors to represent significant differences in demographic variables across buffer zones. Use a clear legend and labels to enhance the map’s effectiveness in conveying your analysis results.
Conclusion
Completing GIS and statistical homework successfully requires a thorough understanding of both disciplines and their integration. By following the strategies outlined in this guide—understanding homework requirements, collecting and preparing data, integrating layers, conducting ANOVA, and customizing maps—you can handle complex assignments with confidence and competence.
Mastering the integration of ANOVA with GIS not only enhances your ability to analyze spatial data but also equips you with valuable skills for data-driven decision-making. These techniques are beneficial not just for academic success but also for future professional endeavors where data analysis and interpretation are crucial. By applying these methods, you'll be well-prepared to tackle challenges and seize opportunities in both academic and professional contexts.