The Role of Statistical Software for Managing and Analyzing Large Datasets
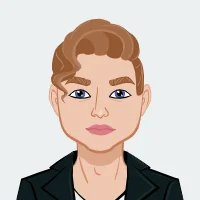
Understanding data analysis and interpreting results is crucial for academic success and practical application across a variety of disciplines. Whether you're studying economics, psychology, business, or any other field where data is important, having a firm grasp of statistical principles is key. This comprehensive guide is designed to provide students with the essential knowledge and skills needed to approach complex statistical assignments with confidence and accuracy.
As you navigate through the world of statistics, you'll encounter a range of concepts and methodologies that will enhance your analytical abilities. This resource covers important areas such as selecting the right statistical tests, conducting bivariate analysis, and interpreting results. Each section provides clear explanations and practical examples to help you understand these fundamental concepts and apply them effectively. Additionally, if you need assistance with specific tasks, such as how to solve your SAS homework, this resource offers insights and techniques to address those challenges efficiently.
Additionally, we will explore the use of advanced statistical software like SAS, which can significantly improve your ability to manage and analyze complex datasets. SAS offers powerful tools to perform a variety of statistical procedures, generate detailed reports, and create visualizations that aid in drawing meaningful conclusions from your data.
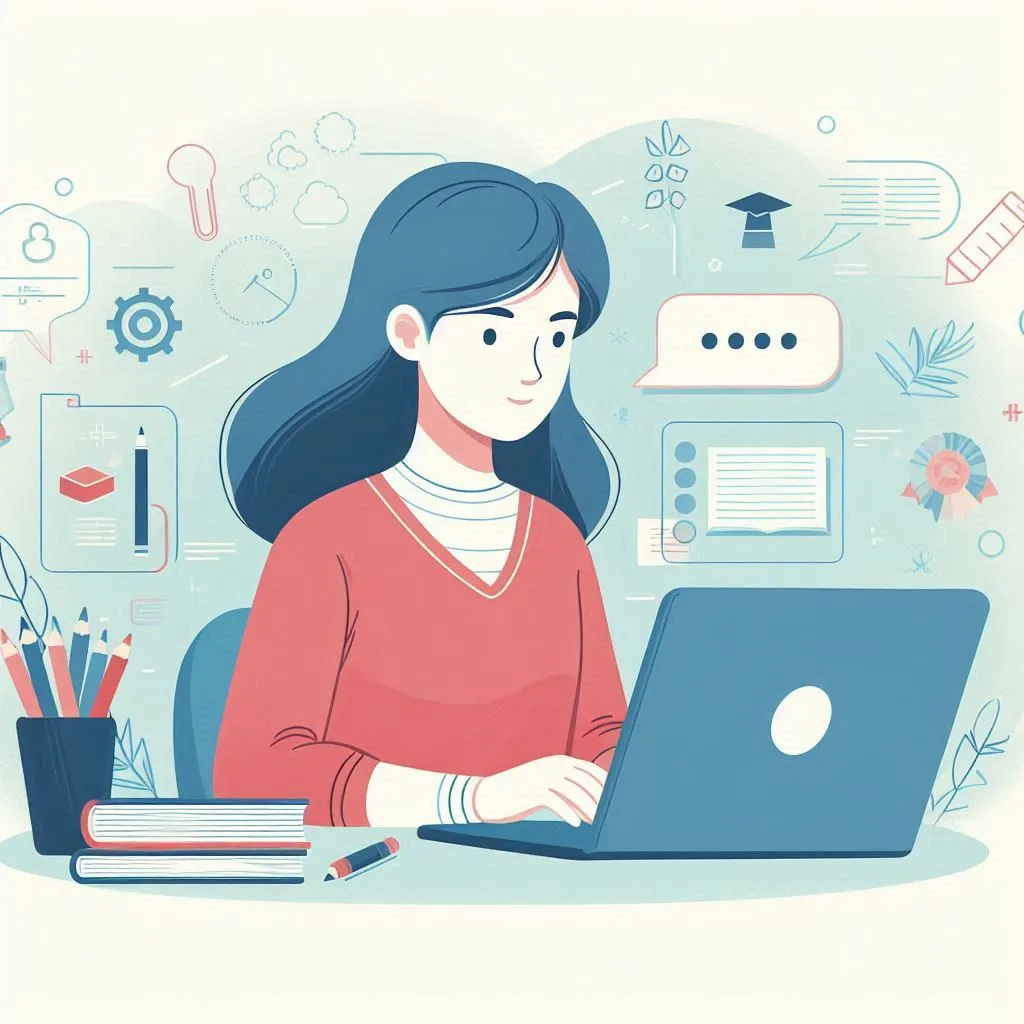
Deciding What Test to Use & Interpreting Results
Choosing the right statistical test is a cornerstone of accurate data analysis. The choice of test hinges on the type of data you have and the specific hypotheses you wish to test. Here’s how to make informed decisions and interpret your results effectively:
- Understanding Hypotheses: Before selecting a test, it’s crucial to define your research hypotheses clearly. Hypotheses can be classified as one-tailed, predicting a specific direction, or two-tailed, testing for effects in both directions. This step is essential as it guides the selection of the appropriate statistical test and ensures that your analysis aligns with your research objectives.
- Determining Levels of Measurement: Identify whether your variables are nominal, ordinal, interval, or ratio. For instance, categorical variables often require chi-square tests, while continuous variables might be analyzed with t-tests or ANOVA. Understanding the level of measurement helps in choosing the correct statistical method and accurately interpreting the results.
- Selecting the Appropriate Test: Based on the nature of your data and hypotheses, choose the statistical test that best fits your needs. Common options include t-tests for comparing means between two groups, ANOVA for comparing means across multiple groups, and regression analysis for exploring relationships between variables. Each test has specific assumptions and requirements that must be met for valid results.
- Interpreting Test Results: After conducting the test, interpret the results by examining p-values, test statistics, and confidence intervals. The p-value indicates the probability of observing the data if the null hypothesis were true, while test statistics provide a measure of the effect size. Confidence intervals offer a range within which the true parameter is likely to lie. Determine if the null hypothesis should be accepted or rejected based on these results and consider the implications of Type I and Type II errors.
- Effect Size and Practical Significance: Beyond statistical significance, assess the effect size to understand the magnitude of the observed effect. Effect size measures, such as Cohen’s d or Pearson’s r, help determine the practical relevance of your findings. Practical significance evaluates whether the observed effect has meaningful implications in real-world contexts, which is crucial for making informed decisions based on your results.
Conducting Bivariate Analysis: A Step-by-Step Guide
Bivariate analysis examines the relationship between two variables, helping you understand how one variable may influence another or how they interact. This analysis is crucial for uncovering patterns, correlations, and potential causal relationships. Follow these steps to conduct bivariate analysis effectively:
- Defining Variables: Clearly define the two variables you are analyzing. Determine their levels of measurement and whether they are independent or dependent. For example, in studying the impact of study time on exam scores, study time is the independent variable, and exam scores are the dependent variable. Properly defining your variables is essential for selecting the appropriate analysis method and accurately interpreting the results.
- Choosing the Analysis Method: Select the appropriate bivariate analysis method based on the nature of your variables. Correlation analysis assesses the strength and direction of the relationship between two continuous variables, while regression analysis predicts values based on the relationship. For categorical variables, chi-square tests or contingency tables may be used to examine associations.
- Collecting and Preparing Data: Gather data on your variables and ensure it is clean and well-organized. This may involve transforming or recoding data to prepare it for analysis. Data preparation is a crucial step to ensure the accuracy and reliability of your results. Ensure that your dataset is complete and free from errors or outliers that could skew the analysis.
- Performing the Analysis: Use statistical software to conduct the analysis. Generate correlation coefficients, regression coefficients, or other relevant statistics depending on your chosen method. Statistical software can handle complex calculations and provide detailed output that aids in interpreting the results.
- Interpreting Results: Analyze the output to determine the strength and significance of the relationship between the variables. Look for patterns, trends, and unexpected findings. Consider the practical implications of your results and how they align with your research hypotheses. Interpreting results involves understanding both statistical significance and practical relevance.
- Reporting Findings: Present your results clearly, including statistical summaries, visualizations, and interpretations. Ensure your report addresses the research questions and provides actionable insights. A well-structured report should include a summary of your findings, visual representations of the data, and a discussion of the implications.
Frequency Distributions & Graphs: Visualizing Data Effectively
Visualizing data through frequency distributions and graphs is essential for understanding and communicating results. Effective data visualization helps in identifying patterns and trends that might not be evident from raw data alone. Here’s how to use these tools effectively:
- Creating Frequency Distributions: Organize data into a frequency distribution table, showing the number of occurrences for each category or range. For example, if analyzing survey responses, list the number of respondents in each satisfaction category. Frequency distributions provide a clear overview of how data is distributed across different categories or intervals.
- Generating Graphs: Use graphs such as bar charts, histograms, or pie charts to visually represent your data. Graphs make it easier to identify patterns, distributions, and trends. Bar charts are useful for comparing categorical data, histograms for continuous data distributions, and pie charts for showing proportions.
- Interpreting Graphs: Examine graphs to identify trends, central tendencies, and variations. Graphs can reveal insights about the data’s distribution and relationships that might not be apparent from numerical summaries alone. Pay attention to any anomalies or outliers that may require further investigation.
- Presenting Data: Incorporate graphs and frequency distributions into your reports to support your analysis. Ensure visualizations are well-labeled, accurate, and easy to interpret. Use clear titles, axis labels, and legends to enhance the readability of your graphs.
Hypothesis Testing: Understanding and Applying Statistical Tests
Hypothesis testing is a critical component of statistical analysis, allowing you to draw meaningful conclusions based on sample evidence. Mastering this process enables you to make informed decisions and validate assumptions with greater accuracy. Here’s a guide to effective hypothesis testing:
- Formulating Hypotheses: Develop null and alternative hypotheses based on your research question. The null hypothesis typically states there is no effect or relationship, while the alternative hypothesis suggests there is an effect or relationship. Formulating clear and testable hypotheses is essential for guiding your analysis and interpreting the results.
- Selecting the Test: Choose a statistical test based on your hypotheses and data types. Options include t-tests for comparing means between two groups, chi-square tests for categorical data, and ANOVA for comparing multiple groups. Each test has specific assumptions and requirements that must be met for valid results.
- Calculating Test Statistics: Perform the test to calculate the test statistic and p-value. The test statistic measures the evidence against the null hypothesis, while the p-value indicates the probability of observing the data if the null hypothesis were true. Calculate these values accurately to determine the significance of your findings.
- Making Decisions: Compare the p-value to your significance level (usually 0.05) to decide whether to accept or reject the null hypothesis. Consider the potential for Type I (false positive) and Type II (false negative) errors in your conclusions. Making informed decisions based on statistical evidence is crucial for drawing valid conclusions.
Effect Size and Practical Significance: Beyond Statistical Significance
Effect size and practical significance provide a deeper understanding of research findings, helping to contextualize statistical results within real-world applications. By evaluating these aspects, you gain insight into the practical implications and relevance of your findings, beyond mere statistical significance. This approach can also be particularly useful when you need to complete your statistics homework, as it helps you understand and apply results in a meaningful way. Here’s how to assess and interpret these important elements effectively:
- Understanding Effect Size: Effect size measures the magnitude of an effect or relationship, offering insight into its practical significance. Common measures include Cohen’s d, Pearson’s r, and eta squared. Understanding effect size helps in interpreting the strength of the observed effect beyond mere statistical significance.
- Interpreting Practical Significance: Assess the practical implications of the effect size. A large effect size indicates a meaningful impact, while a small effect size suggests a less substantial effect. Practical significance evaluates whether the observed effect has real-world relevance or implications for decision-making.
- Contextualizing Results: Consider the effect size in relation to your research question and real-world applications. Evaluate whether the observed effect has practical relevance and how it aligns with your study’s objectives. Contextualizing results helps in understanding the broader implications of your findings.
- Reporting Effect Size: Include effect size measures in your reports to provide a comprehensive view of your findings. Discuss the practical significance and potential impact of the observed effects. Reporting effect size enhances the interpretability and relevance of your results.
Using SAS for Statistical Analysis: Leveraging Software for Efficiency
SAS (Statistical Analysis System) is a powerful tool for conducting statistical analyses and managing data. Leveraging SAS effectively can significantly enhance your efficiency in solving statistical assignments. Here’s how to use SAS to your advantage:
- Understanding SAS Capabilities: Familiarize yourself with SAS’s features, including data management, statistical analysis, and reporting tools. SAS offers a range of procedures for various types of analysis, making it a versatile tool for data analysis. SAS can handle complex data manipulations, perform intricate statistical analyses, and generate detailed reports, making it an indispensable tool for comprehensive statistical analysis.
- Preparing Data in SAS: Import and clean your data using SAS procedures. Ensure your data is well-organized and free of errors before conducting analyses. Data preparation is crucial for accurate and reliable results. Utilize SAS’s data management features to sort, merge, and transform data as needed for your analysis.
- Conducting Analyses: Use SAS procedures to perform statistical tests, generate frequency distributions, and create graphs. SAS provides a range of statistical procedures, including PROC FREQ for frequency distributions, PROC MEANS for descriptive statistics, and PROC REG for regression analysis. Select the appropriate procedures based on your analysis needs.
- Analyzing Output: Examine the output generated by SAS, including statistical summaries, test statistics, and graphs. Use these results to draw conclusions and report your findings. SAS output provides detailed information that aids in understanding and interpreting your data. Pay attention to statistical summaries, such as means and standard deviations, and test statistics, such as t-values and p-values, to draw meaningful conclusions from your analysis.
- Reporting Results: Generate reports using SAS to present your findings clearly and professionally. Include relevant statistics, visualizations, and interpretations in your reports. SAS’s reporting capabilities help in creating well-organized and informative reports. Utilize SAS’s reporting features to customize and format your reports, making them suitable for academic or professional presentations.
Conclusion
Mastering statistical analysis is essential for academic success and the effective communication of research findings. By understanding how to choose the right statistical tests, conduct bivariate analysis, interpret frequency distributions and graphs, and apply hypothesis testing, you can approach your assignments with greater confidence and accuracy. Leveraging software tools like SAS further enhances your ability to analyze data efficiently and effectively. Embrace these concepts and techniques, practice regularly, and you’ll be well-equipped to tackle any statistical challenge that comes your way. With a solid foundation in statistical analysis, you can excel in your coursework, contribute valuable insights to your field of study, and make informed decisions based on data-driven evidence.