Understanding How Symmetric and Skewed Distributions Affect Data Analysis
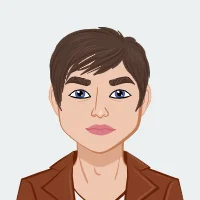
Understanding basic computations and summary statistics is essential for anyone aiming to interpret data effectively and accurately. Mastery of these fundamental skills is crucial not only for completing academic assignments and research projects but also for making informed, data-driven decisions in various professional and real-world contexts. Proficiency in these areas enables individuals to analyze data with confidence, draw meaningful conclusions, and communicate findings clearly, which is indispensable in fields ranging from business and economics to healthcare and social sciences.
Understanding measures of central tendency, such as the mean, median, and mode, helps in identifying the central point around which data values cluster. Measures of spread, like range, variance, and standard deviation, provide insights into the variability or dispersion of the data, allowing you to gauge how spread out the values are from the central point. Measures of location, including percentiles and quartiles, are important for understanding the relative position of data points within a dataset.
These concepts form the backbone of statistical analysis and are integral to various statistical tools and software, such as SAS (Statistical Analysis System). For those struggling with their coursework, SAS homework help can provide crucial support by guiding students through the application of these fundamental computations. With expert assistance, learners can better understand how SAS uses these basic statistics to perform more complex analyses, generate detailed reports, and make informed, data-driven decisions.
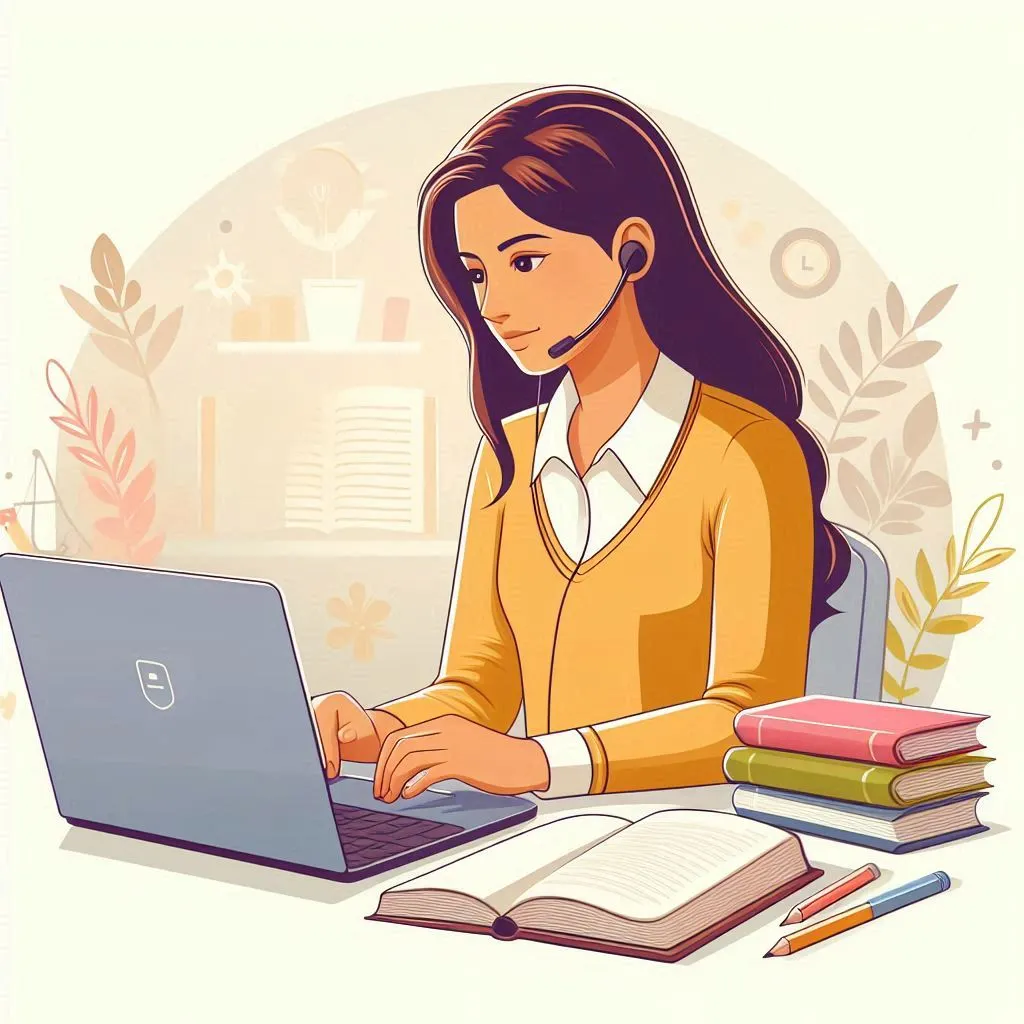
Understanding Measures of Central Tendency
Measures of central tendency are statistical metrics used to identify the center point or typical value of a dataset. They give us a snapshot of where most of the data points lie. The primary measures are the mean, median, and mode.
Mean: The Mathematical Average
The mean, commonly known as the average, is calculated by adding up all the values in a dataset and then dividing by the number of values. It provides a central value that represents the entire dataset.
For example, if you have test scores of 70, 80, 90, and 100, the mean score would be calculated by adding these numbers together (70 + 80 + 90 + 100 = 340) and dividing by the number of scores (4). Thus, the mean score is 340 / 4 = 85.
The mean is a useful measure but can be influenced by extreme values, or outliers. For instance, if one score were 200 instead of 100, the mean would increase significantly, which might not accurately reflect the central tendency of the majority of scores.
Median: The Middle Value
The median is the value that lies in the middle of a dataset when it is ordered from least to greatest. If the number of values is odd, the median is the middle one. If the number of values is even, the median is the average of the two middle values.
In the same dataset of test scores (70, 80, 90, 100), when ordered, the middle values are 80 and 90. The median would be (80 + 90) / 2 = 85.
The median is especially useful when the data has outliers or is skewed, as it provides a better measure of central tendency than the mean in such cases.
Mode: The Most Frequent Value
The mode is the value that appears most frequently in a dataset. A dataset can have one mode, more than one mode, or no mode at all if no value repeats.
For example, in the dataset of test scores (70, 80, 80, 90, 100), the mode is 80, as it appears more frequently than any other value.
While the mode is less commonly used in some analyses, it can be valuable for understanding categorical data or the most common occurrences in a dataset.
Understanding Skewness and the Relationship Between Mean, Median, and Mode
Skewness refers to the asymmetry in the distribution of data values. It indicates whether the data is skewed to the right or left, which impacts how the mean, median, and mode relate to each other.
Right-Skewed Distribution
In a right-skewed distribution, the tail on the right side is longer or fatter than the left side. This means that there are a few high values that pull the mean to the right. In such distributions, the mean is typically greater than the median, and the median is greater than the mode.
For example, income distributions often show right skewness because a small number of people earn significantly more than the majority. As a result, the mean income might be higher than the median, which better represents the typical income of most people.
Left-Skewed Distribution
Conversely, in a left-skewed distribution, the tail on the left side is longer or fatter than the right side. This implies that there are a few low values pulling the mean to the left. Here, the mean is usually less than the median, and the median is less than the mode.
An example of a left-skewed distribution might be the age at which people retire. If most people retire around a certain age but a few retire very early, the mean age of retirement will be lower than the median, reflecting those early retirees.
Symmetric Distribution
A symmetric distribution is one where the left and right sides of the distribution are mirror images of each other. This characteristic implies that the data is evenly distributed around a central point, creating a balanced shape. In such distributions, the mean, median, and mode are all equal or very close to each other, reflecting the central tendency of the data in a uniform manner.
Symmetric distributions are often idealized in statistical analyses and are commonly found in natural phenomena that follow normal distribution patterns. For example, human heights or test scores typically exhibit a bell-shaped curve, where most values cluster around the average, and the frequency of extreme values diminishes symmetrically on either side. This symmetry is crucial in various fields of study, including psychology, education, and biology, as it simplifies the analysis and interpretation of data.
If you find yourself needing assistance to complete your statistics homework, understanding symmetric distributions can be particularly beneficial. This knowledge helps in making accurate predictions and assessing the likelihood of certain outcomes and plays a key role in statistical inference. Many statistical methods and tests assume normality or symmetry in the underlying data distribution, so grasping these concepts is essential for effective analysis and interpretation.
Analyzing Measures of Spread in Data
Measures of spread indicate how much the values in a dataset vary from the central value. Key measures include the range, variance, and standard deviation, which quantify the dispersion and variability within the dataset. These metrics help in understanding the extent of data variability and the consistency of the data distribution.
Range: The Difference Between Extremes
The range is the simplest measure of spread, calculated as the difference between the highest and lowest values in a dataset. It provides a quick sense of how spread out the values are.
For example, in a dataset of ages (22, 25, 30, 35, 40), the range is 40 - 22 = 18 years. This tells us that there is an 18-year difference between the youngest and oldest individuals.
While easy to compute, the range can be affected by outliers. For instance, if one age in the dataset were 100 instead of 40, the range would increase significantly, which might not accurately reflect the overall spread of the majority of ages.
Variance and Standard Deviation: Quantifying Spread
Variance measures the average squared deviation of each value from the mean. It provides a sense of how much the values deviate from the central value. Standard deviation, the square root of the variance, provides a more interpretable measure of spread, as it is in the same units as the data.
For example, in the dataset of test scores (70, 80, 90, 100), calculating variance and standard deviation involves computing how much each score deviates from the mean score of 85 and averaging those deviations.
A low standard deviation indicates that the data points are close to the mean, suggesting consistency. A high standard deviation means the data points are spread out over a wider range, indicating variability.
Measures of Location: Percentiles, Quartiles, and Z-Scores
Measures of location help you understand the position of specific values within a dataset. They include percentiles, quartiles, and z-scores.
Percentiles: Dividing Data into Parts
Percentiles divide a dataset into 100 equal parts. The nth percentile is the value below which n percent of the data falls. For example, the 25th percentile (or first quartile) is the value below which 25% of the data points lie.
Percentiles are useful for understanding the relative standing of a data point within the distribution. For instance, if a student's test score is at the 90th percentile, it means the student scored better than 90% of their peers.
Quartiles: Dividing Data into Four Parts
Quartiles are specific percentiles that divide the data into four equal parts. The first quartile (Q1) is the 25th percentile, the second quartile (Q2) is the 50th percentile (median), and the third quartile (Q3) is the 75th percentile.
Quartiles are often used to understand the spread and central tendency of the middle 50% of the data. For example, in salary data, the quartiles can help identify the range of salaries where most of the employees fall.
Z-Scores: Standardizing Data
Z-scores standardize data by measuring how many standard deviations a value is from the mean. A z-score of 0 indicates that the value is exactly at the mean, while positive or negative values indicate how many standard deviations the value is above or below the mean.
Z-scores are useful for comparing data points across different datasets. For example, if two students have test scores from different exams, converting their scores to z-scores allows for a meaningful comparison of their relative performance.
Implementing SAS for Effective Data Analysis
SAS (Statistical Analysis System) is a powerful software suite used for advanced analytics, multivariate analysis, business intelligence, and more. While the focus here is on the concepts rather than the code, understanding how SAS integrates these statistical measures can enhance your analysis.
- Importing Data: SAS supports various data formats and sources. Importing data into SAS is the first step in any analysis process. Whether using CSV files, Excel spreadsheets, or databases, SAS provides tools for data import and management.
- Descriptive Statistics: SAS offers procedures to calculate descriptive statistics like mean, median, mode, variance, and standard deviation. By using built-in procedures, you can easily compute these statistics and generate reports.
- Visualizations: SAS provides graphical tools for data visualization, including histograms, box plots, and scatter plots. These visualizations help you understand the distribution and spread of your data more intuitively.
- Advanced Analysis: For more complex analyses, SAS offers advanced statistical techniques and modeling tools. These include regression analysis, hypothesis testing, and more, enabling in-depth examination of your data.
Conclusion
Understanding basic computations and summary statistics is essential for any student or researcher involved in data analysis. Mastering these concepts allows you to summarize, interpret, and make decisions based on your data effectively. SAS, with its comprehensive suite of tools, simplifies the process of calculating and interpreting these statistics, making it an invaluable resource for statistical analysis. Whether you're preparing for an assignment, conducting research, or analyzing data for a project, the concepts and techniques discussed in this guide will help with data analysis homework tasks, enabling you to navigate the complexities of data analysis with confidence. By leveraging SAS, you can efficiently perform statistical computations, visualize your data, and gain deeper insights into your analysis. In summary, mastering basic computations and summary statistics is a crucial step in becoming proficient in data analysis. With the knowledge of key concepts and the power of SAS, you can confidently tackle any statistical challenge and uncover the valuable insights hidden within your data."